Global coupled atmosphere-ocean general circulation models
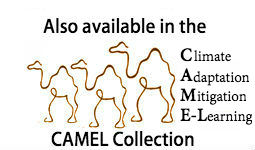
This is Section 4.2 of the Arctic Climate Impact Assessment.
Lead Authors: Vladimir M. Kattsov, Erland Källén; Contributing Authors: Howard Cattle, Jens Christensen, Helge Drange, Inger Hanssen-Bauer,Tómas Jóhannesen, Igor Karol, Jouni Räisänen, Gunilla Svensson, Stanislav Vavulin; Consulting Authors: Deliang Chen, Igor Polyakov, Annette Rinke
The atmosphere (Atmosphere layers), oceans, land surface, cryosphere, and associated biology and chemistry form interactively coupled components of the total climate system. Climate models are primary tools for the study of climate, its sensitivity to external and internal forcing factors, and the mechanisms of climate variability and change. These models attempt to take into account the various processes important for climate in the atmosphere, the oceans, the land surface, and the cryosphere, as well as the interactions between them (Fig. 4.1). In addition, models are increasingly incorporating components that describe the role of the biosphere and chemistry in order to provide a comprehensive description of the total earth system. Because physical processes and feedbacks play a key role in the arctic climate system, this section focuses primarily on the physical components of climate models. However, land-surface biology is an important factor in determining the key thermal and radiative properties of the land surface, surface hydrology, turbulent heat and gas exchanges, and other processes. Likewise, the interaction of ocean biology with physical processes is important for air-sea gas exchange, including key processes related to cloud formation such as dimethyl sulfide exchange.
Coupled AOGCMs are made up of component models of the atmosphere, ocean, cryosphere, and land surface that are interactively coupled via exchange of data across the interfaces between them. For example, the ocean component is driven by the atmospheric fluxes of heat, momentum, and freshwater simulated by the atmospheric component. These heat and freshwater fluxes are themselves functions of the sea surface temperatures simulated by the ocean model. Other driving fluxes for the ocean are produced by the brine rejection that occurs during sea-ice formation, freshwater from sea-ice melt, and freshwater river discharge at the continental boundaries.
Atmosphere-ocean general circulation models are continually evolving. The state-of-the-art climate modeling described in this section refers to the generation of models from the late 1990s and very early 2000s, and is close to that evaluated by the IPCC[1].
Contents
- 1 Equilibrium and transient response experiments (4.2.1)
- 2 Initialization and coupling issues (4.2.2)
- 3 Atmospheric components of AOGCMs (4.2.3)
- 4 Ocean components of AOGCMs (4.2.4)
- 5 Land-surface components of AOGCMs (4.2.5)
- 6 Cryospheric components of AOGCMs (4.2.6)
- 7 AOGCMs selected for the ACIA (4.2.7)
- 8 Summary (4.2.8)
- 9 References
- 10 Citation
Equilibrium and transient response experiments (4.2.1)
Early climate simulations were conducted using atmospheric models coupled to highly simplified representations of the ocean. In these models, only the upper ocean was normally represented and then only as a simple fixed-depth slab of water some tens of meters deep in which the temperature responded directly to changes in atmospheric heat fluxes. Such models are still useful for short sensitivity experiments, such as exploring the impact of new representations of physical processes on climate change in experiments in which the concentration of atmospheric GHGs is instantaneously doubled in the model atmosphere. These models enable a quick assessment of the "equilibrium response" of climate to a given perturbation. The equilibrium response is the change in climate resulting from a perturbation (e.g., a specified increase in effective carbon dioxide (CO2) concentration) after a period long enough for the climate to reach an equilibrium state. However, such models assume that vertical and horizontal heat transports in the ocean do not change when the climate changes.
Many centers have developed models with full dynamic deep-ocean components over the past decade. The dynamic oceans introduce the long timescales (multicentury to millennia) associated with the equilibration of the abyssal ocean. Such long timescales are absent in the models that represent the ocean as a shallow slab of water. In particular, this development has enabled the exploration of the "transient response" of climate to changing concentrations of GHGs, as well as the examination of many aspects of natural climate variability. The transient response is the change over time as the perturbation (e.g., a continuous change in GHG concentrations) is applied. In the case of GHG-induced temperature change, the transient response is smaller than the equilibrium response because the large thermal inertia of the oceans slows the rate of warming.
Initialization and coupling issues (4.2.2)
Owing to embodied feedbacks between ocean and atmosphere, an AOGCM-simulated climate is less constrained than climates simulated by stand-alone atmospheric or oceanic general circulation models (GCMs). Upon coupling, an AOGCM-simulated climate typically undergoes a so-called coupling shock (fast drift due to imbalances in the initial conditions between the component models at the time of coupling) and then, after a close-to-balance state between the interacting components of the AOGCM has been achieved, a gradual drift toward the model’s equilibrium climatic state. The presence of climate drift, if it is significant, can complicate the study of a possible climate change signal. For example, large drifts can potentially distort the behavior of various feedback processes present in the climate system and, dependent on the mean state of the model, distort the calculated climatic response to a given change in forcing.
The climate drift problem can introduce many technical considerations into the application of AOGCMs. To limit the influence of climate drift (especially the fast-drift component), careful initialization of AOGCMs is very important. This has led to a relatively wide array of initialization methods[2]. Initialization techniques often include a sequence of runs of component models separately, and in the coupled mode the components are constrained by observations at their interfaces. This makes it possible to reduce the climate drift and, particularly, the coupling shock.
Until recently, it has been necessary to use so-called "flux adjustments" (or "flux corrections"[3]) to prevent drift in the climate of the coupled system that arises from inadequacies in the component models and in the simulated fluxes at their interfaces. These adjustments are normally derived as fields of spatially varying "corrections" to the heat and freshwater fluxes between the atmosphere (Atmosphere layers) and ocean components of the model. They are often derived during a calibration run of the AOGCM in which the sea surface temperatures and surface salinities are constrained to observed climatological values of these quantities. The flux adjustments are then applied to succeeding runs of the model to provide improved simulation of the coupled system. In some cases, flux adjustments have also been applied to momentum fluxes. While flux adjustments have not been applied over land, it has in the past been necessary to flux-adjust the fields of sea-ice concentration and thickness. A driver in the development of coupled models has been to improve models to the stage where they can run without flux adjustments, as is now the case for some AOGCMs. In the AOGCMs that continue to use this technique, flux adjustments have become smaller as models have improved. Interestingly, the IPCC did not find systematic differences in the simulation of internal climate variability between flux-adjusted and non-flux adjusted AOGCMs[4], thus supporting the use of both types of model in the detection and attribution of climate change.
Atmospheric components of AOGCMs (4.2.3)
The atmospheric component of AOGCMs enables simulation of the evolution with time of the spatial distributions of the vector wind, temperature, humidity, and surface pressure. This is done by discretization of the basic equations governing the behavior of the atmosphere, and implementation of these discretized equations on an appropriate computer. The equations are time-stepped forward at intervals that typically vary from a few minutes to tens of minutes, depending on the model formulation and resolution, to produce an evolving simulation of the behavior of the atmospheric flow and associated temperature, humidity, and surface-pressure fields.
The model dynamics are usually represented either as periodic functions defined as the sum of several waves (spectral models) or on a grid of points (finite-difference models) covering the globe for various levels of the atmosphere. Typically, the atmospheric components of the generation of climate models evaluated by the IPCC[5] operated on grids with a horizontal spacing of 200 to 300 km and 10 to 20 vertical levels. Various schemes are available for the specification of vertical coordinates[6].
Simulation of some climatic variables in high latitudes (e.g., atmospheric moisture) using global models presents certain problems. Finite-difference GCMs require undesirable filtering operations in order to avoid computational instability when a reasonable time step is used in regions of converging meridians such as the Arctic. While polar filtering is not needed in spectral models, these models produce fictitious negative moisture amounts in the dry high-latitude atmosphere (Atmosphere layers), thus calling for correction procedures. Both problems are apparently overcome by the application of semi-Lagrangian schemes for moisture advection, which are used in a number of atmospheric general circulation models (AGCMs). However, in semi-Lagrangian schemes, the advantages of large time steps and the absence of spurious negative moisture values are partially offset by the lack of exact moisture conservation. New schemes have recently started to appear that combine the semi-Lagrangian approach with mass conservation (e.g., Zubov et al., 1999), but have other disadvantages. Hopes for improved climate simulations of the polar regions are also associated with spherical geodesic grids, which allow for approximately uniform discretization of the sphere. Such grids are already used in some global numerical weather-prediction models[7].
A key issue is the simulation of the basic physical processes that take place in the atmosphere and determine many of the feedbacks for climate variability and change. Examples include the representation of clouds and radiation; dry and moist convective processes; the formation of precipitation and its deposition on the surface as rain or snow; the interactions between the atmosphere and the land-surface orography (including the drag on the atmosphere caused by breaking gravity waves); and atmospheric boundary-layer processes and their interaction with the surface. Because these processes take place on scales much smaller than the model grid, they must be represented in terms of the large. scale variables in the model (vector wind, temperature, humidity, and surface pressure). Key atmospheric processes from an arctic surface climate perspective include the representations of the planetary boundary layer, clouds, and radiation.
Energy, momentum, and moisture from the free troposphere (Atmosphere layers) are transferred via the atmospheric boundary layer (ABL) to the surface and vice versa. Atmospheric general circulation models have difficulty with the proper representation of turbulent mixing processes in general, which has implications for the representation of boundary-layer clouds[8]. The ABL in the Arctic differs significantly from its mid-latitude counterpart, so parameterizations based on mid-latitude observations tend to perform poorly in the Arctic. Parameterizations of the surface fluxes are usually based on the Monin-Obukhov similarity theory. These parameterizations work reasonably well for cases where the vertical stratification of the atmosphere is weakly stable, but simulate surface fluxes of momentum, heat, and water vapor that are too small in the very stable stratified conditions[9] common in the high Arctic. In the very stable cases, turbulence may not be stationary, local, and continuous[10] – assumptions used in ABL parameterizations of surface fluxes. In addition, vertical resolution is a critical issue because the very thin stable surface layer is usually shallower than the first vertical model layer. Deviations from observations in the ABL during winter, found in simulations with a regional climate model for the Arctic (Section 4.5.1 (Global coupled atmosphere-ocean general circulation models)), indicate the necessity of improvements in the atmospheric parameterization that better describe the vertical stratification and atmosphere–surface energy exchange[11]. The mean monthly turbulent heat-flux distribution (Global surface temperature distribution) at the surface strongly depends on different ABL parameterizations and leads to different spatial distributions of temperature, wind, moisture, and other variables throughout the arctic atmosphere. The greatest changes are found in the ABL above the sea-ice edge in January.
Model resolution, both horizontal and vertical, is a problem in simulating the arctic ABL. The vertical discretization of current AGCMs cannot resolve the large temperature gradients and inversions that exist in the arctic ABL. Insufficient resolution gives rise to sensible heat fluxes in the models that tend to be too large. However, simply increasing the resolution will not solve the problem. Even if the very stable ABL can be simulated in finer detail, the fundamental problem of current theories predicting turbulent fluxes that are too small will still remain.
Specific cloud types observed in the arctic ABL present a serious challenge for atmospheric models. Parameterizing low-level arctic clouds is particularly difficult because of complex radiative and turbulent interactions with the surface[12].
The atmospheric components of AOGCMs usually focus on representation of tropospheric processes and the effects of stratospheric processes on the troposphere, while their descriptions of stratospheric processes are less satisfactory. For example, the insufficient vertical resolution in the stratosphere (as compared to that in the troposphere) prevents the atmospheric components of AOGCMs from properly representing important stratospheric phenomena, such as the quasi-biennial oscillation and sudden stratospheric temperature increases[13].
Current AOGCMs generally do not include interactive atmospheric chemistry models[14]. Most of the atmospheric photochemical processes are therefore simulated with chemical transport models (CTMs) that use atmospheric wind velocities and temperature prescribed either from observational data or from GCM simulations. In the latter case, CTMs can be used to project the evolution of the atmospheric content of ozone, other radiatively active gases (e.g., methane and nitrous oxide), and aerosols[15]. Projections of the distributions of tropospheric ozone and aerosols (sulfates, soot, sea salt, and mineral dust collectively known as "arctic haze") are particularly important to climate change projections[16].
Ocean components of AOGCMs (4.2.4)
The oceanic component in AOGCMs has improved substantially over the past decade. These models now include representation of the full dynamics and thermodynamics of the global ocean basins and allow simulation of the full three-dimensional current, temperature, and salinity structure of the ocean and its evolution. Important physical processes are associated with the upper-ocean mixed layer and diffusive processes in the ocean. The freezing, melting, and dynamics of sea ice and ice–ocean interactions are also taken into account. Until recently, because of limitations in available computing power, AOGCMs typically had similar horizontal resolution in the ocean and atmospheric components. Such ocean models poorly represent the large-scale ocean current structure, not only because of the lack of resolution of narrow boundary currents such as the Gulf Stream and the Kuroshio, but also because of the high viscosity coefficients necessary for computational stability[17]. However, as available computing power has increased, the resolution of the ocean component of AOGCMs has increased to roughly one degree of latitude and longitude. Although this resolution does not allow explicit representation of ocean eddies (a resolution of one-third of a degree is considered "eddy permitting", and one-ninth of a degree or better, "eddy-resolving"), it does result in a much-improved representation of ocean current structure.
The Arctic Ocean has always been and still remains one of the weak spots in AOGCMs. This is partly due to specific numerical problems such as the singularity of the longitude-latitude spherical coordinates (converging meridians) at the North Pole[18].
Until recently, filtering, or even inserting an artificial island at the North Pole, have been among the usual, but undesirable, ways to overcome the pole problem. Rotating grids or introducing alternative grids, for example, geodesic grids providing approximately uniform discretization of the sphere[19] or using curvilinear generalized coordinates[20], are now being pursued in order to eliminate the converging meridian problem. Such features are now starting to appear in oceanic components of AOGCMs[21]. However, a greater challenge is insufficient understanding of some phenomena related to the general circulation of the Arctic Ocean and subarctic seas. In particular, improvement is needed in representing ocean/atmosphere (Atmosphere layers)/sea-ice interaction processes in order to better evaluate their importance within the context of natural variability and anthropogenically forced change in the climate system. A particular problem for the oceanic component of AOGCMs is the treatment of air–ice–ocean interactions and water-mass formation (creation of water bodies with a homogenous distribution of temperature and salinity) over the shallow continental shelves, which requires adequate resolution of shallow water layers, water-mass formation and mixing processes, continental runoff, and ice processes.
Land-surface components of AOGCMs (4.2.5)
The land-surface components of climate models include representation of the thermal and soil-moisture storage properties of the land surface through modeling of its upper layers. Key properties include surface roughness and albedo, which are normally specified from global datasets, although models with interactive land-surface properties are now being developed.
Possible changes in vegetation and the effects that these changes may have on future climate are not often taken into account in climate change projections. These effects may be substantial and would be manifested in the local fluxes of water, heat, and momentum controlled by surface roughness, albedo, and surface moisture. The arctic land types have special features that are not well represented in the present generation of climate models[22]. This is particularly true for winter conditions where snow distribution and its interaction with vegetation are poorly understood and modeled.
The discharge of river water to the ocean, especially to the Arctic Ocean whose freshwater budget is much more influenced by terrestrial water influx than are the budgets of other oceans, is of potential importance to climate change. The land-surface components of AOGCMs usually include river-routing schemes, in which the land surface is represented as a set of watersheds draining the runoff (integrated over their territories at each time step) into the grid boxes of the ocean model closest to the grid points specified as river mouths in the land-surface model. Such schemes are able to provide reasonable annual means of the discharge, but shift and sharpen its seasonal cycle, especially for the Arctic Ocean terrestrial watersheds with their high seasonality of discharge. More comprehensive river-routing schemes[23], allowing for simulations of horizontal transport of the runoff within model watersheds, are usually not used interactively in AOGCMs.
Cryospheric components of AOGCMs (4.2.6)
Snow cover and sea ice are the two primary elements of the cryosphere represented interactively in AOGCMs, although some models now incorporate explicit parameterizations of permafrost processes. The large ice sheets (Glaciers and ice sheets in the Arctic) are represented, although non-interactively, by land-surface topography and surface albedo (typically fixed at a value of around 0.8). Likewise, there is usually no explicit representation of glaciers.
The insulating effects and change in surface albedo due to snow cover are of particular importance for climate change projections. AOGCMs demonstrate varying degrees of sophistication in their snow parameterization schemes. For example, some can represent snow density, liquid water storage, and wind-blown snow[24]. Advanced albedo schemes incorporate dependencies on snow age or temperature. However, a major uncertainty exists regarding the ability of AOGCMs to simulate terrestrial snow cover[25] (Section 6.4 (Global coupled atmosphere-ocean general circulation models)), particularly its albedo effects and the masking effects of vegetation that are potentially important in determining the surface energy budget.
Sea-ice components of AOGCMs usually include parameterizations of the accumulation and melting of snow on the ice, and thermodynamic energy transfers between the ocean and atmosphere (Atmosphere layers) through the ice and snow. Most of the AOGCMs evaluated by the IPCC[26] employed simplistic parameterizations of sea ice. Recent advances in stand-alone sea-ice modeling, including those in modeling sea-ice thermodynamics (e.g., introducing the effects of subgrid-scale parameterizations with multiple thickness categories – the so-called "ice-thickness distribution"), are now being incorporated into AOGCMs. However, understanding is still insufficient for treating some atmosphere–ice–ocean interaction issues (e.g., heat distribution between concurrent lateral and vertical ice melt or accumulation). The primary differences among the various representations relate to treatment of internal stresses in calculating sea-ice model dynamics. An evaluation of the different treatment of sea-ice rheologies (relationships between internal stresses and deformation) was the core task for the Sea-Ice Model Intercomparison Project (SIMIP) initiated in the late 1990s. Having considered a hierarchy of stand-alone sea-ice models with different dynamic parameterizations, SIMIP found the viscous-plastic rheology to provide the best simulation results and adopted it as a starting point for further optimizations[27]. Other developments, including the elastic-viscous-plastic rheology[28], are helpful in acheiving high computational efficiency. However mature the status of stand-alone sea-ice dynamics modeling, some AOGCMs still employ a simple, so-called "free drift" scheme that only allows ice to be advected with ocean currents. There is a large range later in the ability of AOGCMs to simulate the position of the ice edge and its seasonal cycle[29]. However, there is no obvious connection between the fidelity of simulated ice extent and the inclusion of an ice-dynamics scheme. This is apparently due to the additional impact of simulated wind-field errors[30], which may offset, improvements from the inclusion of more realistic ice dynamics. Conversely, the importance of improved sea-ice dynamics and thermodynamics has become apparent, and the AOGCM community is responding by including more sophisticated treatments of sea-ice physics.
AOGCMs selected for the ACIA (4.2.7)
Selecting AOGCM simulation results to be used in an impact assessment is not a trivial task, given the variety of models. The IPCC[31] concluded that the varying sets of strengths and weaknesses that AOGCMs display means that, at this time, no single model can be considered "best" and it is important to utilize results from a range of coupled models in assessment studies. The choice of AOGCMs for this assessment used the criteria suggested by Smith et al.[32]: vintage, resolution, validity, representativeness of results, and accessibility of the model outputs.
While models do not necessarily improve with time, later versions (often with higher resolution) are usually preferred to earlier ones. An important criterion for selecting an AOGCM to be used in constructing regional climate scenarios is its validity as evaluated by analyses of its performance in simulating present-day and past climates (the evolution of 20th century climate in particular). The validity is evaluated by comparing the model output with observed climate, and with output from other models for the region of interest and larger scales, to determine the ability of the model to simulate large-scale circulation patterns. Well-established systematic comparisons of this type are provided by international model intercomparison projects (MIPs, see Box 4.2). Finally, when several AOGCMs are to be selected for use in an impact assessment, the model results should span a representative range of changes in key variables in the region under consideration.
Box 4.2. Model intercomparison projects Model intercomparison projects (MIPs) allow comparison of the ability of different models to simulate current and perturbed climates, in order to identify common deficiencies in the models and thus to stimulate further investigation into possible causes of the deficiencies[33].This is currently the only way to increase the credibility of future climate projections. Participation in MIPs is an important prerequisite for an AOGCM to be employed in constructing climate scenarios (e.g., for the ACIA). In MIPs, models of the same class (AOGCMs, stand-alone AGCMs or oceanic GCMs, RCMs) are run for the same period using the same forcings.Typically, diagnostic subprojects are established that concentrate upon analyses of specific variables, phenomena, or regions. Occasionally, experimental subprojects are initiated, aimed mainly at answering questions related to model sensitivity. Of the many international MIPs conducted in the past decade, two are of primary importance for the ACIA: the Atmospheric Model Intercomparison Project (AMIP)[34], and the Coupled Model Intercomparison Project (CMIP)[35]. Both included subprojects devoted specifically to model performance at high latitudes among their numerous diagnostic subprojects. Thirty AGCMs were included in the second phase of the AMIP (AMIP-II, concluded in 2002). All of these were forced with the same sea surface temperatures (SSTs) and sea-ice extents prescribed from observations, and a set of constants, including GHG concentrations.The AMIP-II simulations span the period from 1979 to 1996. AMIP findings related to AGCM performance in the Arctic have been reported since the early 1990s[36]. Coupled Model Intercomparison Project experiments belong to the class of idealized (e.g., 1% per year increase in CO2) transient experiments with AOGCMs. Räisänen (2001) discussed some results of the second phase of the CMIP (CMIP2) related to the Arctic (see also Section 4.4.5 (Global coupled atmosphere-ocean general circulation models)). The Climate of the 20th Century project was initiated in order to determine to what extent stand-alone AGCMs are able to simulate observed climate variations of the 20th century against a background of natural variability[36]. In this MIP, the AGCMs are forced with observed SSTs and sea-ice extents and prescribed changes in radiative forcing (GHGs, trace gases, stratospheric and tropospheric ozone, direct and indirect effects of sulfate aerosols, solar variations, and volcanic aerosols). The outputs of models archived at the IPCC Data Distribution Center provide an additional opportunity for AOGCM intercomparison[37].The archived outputs have a limited set of variables, but include at least two scenarios (A2 and B2) from the IPCC Special Report on Emissions Scenarios (SRES)[38] and at least two pre-SRES (IS92a) emissions scenarios (GHGs only and GHGs plus sulfate aerosols). The simulation results that are available usually span the 20th and 21st centuries.The selection of these AOGCMs by the IPCC for use in its Third Assessment Report[39] was an indication that these models provide the most viable basis for climate change assessment. The foci of the Arctic Regional Climate Model Intercomparison Project (ARCMIP) include the surface energy balance over ocean and land, clouds and precipitation processes, stable planetary boundary layer turbulence, icealbedo feedback, and sea-ice processes[40]. Another international effort – the Arctic Ocean Model Intercomparison Project (AOMIP) – aims to identify strengths and weaknesses in Arctic Ocean models using realistic forcing[41].The major goals of the project are to examine the ability of Arctic Ocean models to simulate variability at seasonal to decadal scales, and to qualitatively and quantitatively understand the behavior of the Arctic Ocean under changing climate forcing. Other GCM MIPs of relevance to the ACIA include the Ocean Model Intercomparison Project[42], which is designed to stimulate the development of ocean models for climate research, and the Paleoclimate Modeling Intercomparison Project[43], which compares AGCM/OUML models (see Box 4.1) and AOGCMs in simulations of paleoclimate conditions during periods that were significantly different from the present-day climate.There are also a number of MIPs devoted to intercomparison of specific parameterizations employed in GCMs, including the Sea-Ice Model Intercomparison Project[44], the Snow Models Intercomparison Project[45], and polar clouds[46]. |
Section 1.4.2 (Global coupled atmosphere-ocean general circulation models) provides details of the procedure for selecting AOGCMs for the ACIA. Initially, a set of the most recent and comprehensive AOGCMs whose outputs were available from the IPCC Data Distribution Center was chosen. This set was later reduced to five AOGCMs (two European and three North American), primarily due to the accessibility of model output, as well as storage and network limitations. By the initial phase of the ACIA, at least one Special Report on Emissions Scenarios (SRES)[47] B2 simulation (see Section 4.4.1 (Global coupled atmosphere-ocean general circulation models)) extending to 2100 had been generated by each of the ACIA-designated models. All of the models are well documented, participate in major international MIPs, and have had their pre-SRES simulations analyzed for the Arctic and the results published[48]. The five ACIA-designated models listed in Table 4.1, together with information on their formulations, provided the core data for constructing the ACIA climate change scenarios.
Table 4.1. Key features of the ACIA-designated AOGCMs. | ||||||
Atmospheric resolutiona |
Ocean resolutionb |
Land-surface schemec |
Sea-ice modeld |
Flux adjustmente |
Primary Reference | |
CGMC2 |
||||||
Canadian Centre for Climate Modeling and Analysis, Canada |
T32 (3.8° x 3.8°) L10 |
1.8° x 1.8° L29 |
M, BB, F, R |
T, R |
H, W |
|
CSM_1.4 |
||||||
National Center for Atmospheric Research, US |
T42 (2.8° x 2.8°) L18 |
2.0° x 2.4° L45 |
C, F |
T, R |
||
ECHAM4/OPYC3 |
||||||
Max-Planck Institute for Meteorology, Germany |
T42 (2.8° x 2.8°) L19 |
2.8° x 2.8° L11 |
M, BB, R |
T, R |
T*, W* |
|
GFDL_R30_c |
||||||
Geophysical Fluid Dynamics Laboratory, U.S. |
R30 (2.25° x 3.75°) L14 |
2.25° x 1.875° |
B, R |
T, F |
H, W |
|
HadCM3 |
||||||
Hadley Centre for Climate Prediction and Research, U.K. |
2.5° x 3.75° |
1.25° x 1.25° |
C, F, R |
T, F |
- |
|
(a) Horizontal resolution is expressed either as degrees latitude by longitude or as a spectral truncation (either triangular (T) or rhomboidal (R)) with a rough translation to degrees latitude and longitude. Vertical resolution (L) is the number of vertical levels; (b) Horizontal resolution is expressed as degrees latitude by longitude, while vertical resolution (L) is the number of vertical levels; (c) B=standard bucket hydrology scheme (single-layer reservoir of soil moisture which changes with the combined action of precipitation (snowmelt) and evaporation, and produces runoff when the water content reaches the prescribed maximum value); BB=modified bucket scheme with spatially varying soil moisture capacity and/or surface resistance; M=multilayer temperature scheme; C=complex land-surface scheme usually including multiple soil layers for temperature and moisture, and an explicit representation of canopy processes; F=soil freezing processes included; R=river routing of the discharge to the ocean (land surface is represented as a set of river drainage basins); (d) T=thermodynamic ice model; F="free drift" dynamics; R=ice rheology included; (e) H=heat flux; W=freshwater flux; asterisks indicate annual mean flux adjustment only. |
Summary (4.2.8)
Atmosphere (Atmosphere layers)-ocean general circulation models are widely acknowledged to be the primary tool for projecting future climate. As understanding of the earth’s climate system increases and computers become more sophisticated, the scope of processes and feedbacks simulated by AOGCMs is steadily increasing. In addition to representing the general circulation of the atmosphere and the ocean, the AOGCMs include interactive components representing the land surface and cryosphere. The biosphere and the carbon (Carbon cycle) and sulfur cycle components of AOGCMs are evolving, while the atmospheric chemistry component is currently being developed offline. The ability to increase confidence in model projections of arctic climate is limited by the need for further advances in the representation of the arctic climate system in the AOGCMs.
Chapter 4: Future Climate Change: Modeling and Scenarios for the Arctic
4.1. Introduction (Global coupled atmosphere-ocean general circulation models)
4.2. Global coupled atmosphere-ocean general circulation models
4.3. Simulation of observed arctic climate with the ACIA designated models
4.4. Arctic climate change scenarios for the 21st century projected by the ACIA-designated models
4.5. Regional modeling of the Arctic
4.6. Statistical downscaling approach and downscaling of AOGCM climate change projections
4.7. Outlook for improving climate change projections for the Arctic
References
- Template:Note.g., Bitz, C., G. Flato and J. Fyfe, 2002. Sea ice response to wind forcing from AMIP models. Journal of Climate, 15:523–535;-- Frei, A., J.A. Miller and D.A. Robinson, 2003a. Improved simulations of snow extent in the second phase of the Atmospheric Model Intercomparison Project (AMIP-2). Journal of Geophysical Research, 108:(D12), 4369, doi:10.1029/2002JD003030;-- Kattsov,V.M.,V.P. Meleshko,V.M. Gavrilina,V.A. Govorkova and T.V. Pavlova, 1998. Freshwater budget of the polar regions as simulated with current atmospheric general circulation models. Izvestia Russian Academy of Sciences Phys. Atmos. Ocean, 34:479–489.-- Kattsov,V.M., J.E.Walsh, A. Rinke and K. Dethloff, 2000. Atmospheric climate models: simulations of the Arctic Ocean fresh water budget components. In: E.L. Lewis, E.P. Jones, P. Lemke, T.D. Prowse and P.Wadhams (eds.). The Freshwater Budget of the Arctic Ocean, pp. 209–247. Kluwer Academic Publishers;-- Tao, X., J.E.Walsh and W.L. Chapman, 1996. An assessment of global climate model simulations of Arctic air temperatures. Journal ofClimate, 9:1060–1076.;-- Walsh, J.E.,V. Kattsov, D. Portis and V. Meleshko, 1998. Arctic precipitation and evaporation: model results and observational estimates. Journal of Climate, 11:72–87.-- Walsh, J.E.,V. Kattsov,W. Chapman,V. Govorkova and T. Pavlova, 2002. Comparison of Arctic climate simulations by uncoupled and coupled global models. Journal of Climate, 15:1429–1446.
Citation
Committee, I. (2012). Global coupled atmosphere-ocean general circulation models. Retrieved from http://editors.eol.org/eoearth/wiki/Global_coupled_atmosphere-ocean_general_circulation_models- ↑ IPCC, 2001. Climate Change 2001: The Scientific Basis. Contribution of Working Group I to the Third Assessment Report of the Intergovernmental Panel on Climate Change. J.T. Houghton, Y. Ding, D.J. Griggs, M. Noguer, P.J. van der Linden, X. Dai, K. Maskell and C.A. Johnson (eds.). Intergovernmental Panel on Climate Change. Cambridge University Press, 881pp.
- ↑ Stouffer, R.J. and K.W. Dixon, 1998. Initialization of coupled models for use in climate studies. In: A. Staniforth (ed.). Research Activities in Atmospheric and Oceanic Modelling, pp. I.1–I.15. Rep. 27, WMO/TD-No. 865.
- ↑ Sausen, R., K. Barthel and K. Hasselmann, 1987. A flux correction method for removing the climate drift of climate models. Report No. 1. Max Planck Institute for Meteorology, 39pp.
- ↑ McAvaney, B.J., C. Covey, S. Joussaume, V. Kattsov, A. Kitoh, W. Ogana, A.J. Pitman, A.J. Weaver, R.A. Wood and Z.-C. Zhao, 2001. Model evaluation. In: J.T. Houghton, Y. Ding, D.J. Griggs, M. Noguer, P.J. van der Linden, X. Dai, K. Maskell and C.A. Johnson (eds.). pp. 471–524. Climate Change 2001: The Scientific Basis. Contribution of Working Group I to the Third Assessment Report of the Intergovernmental Panel on Climate Change. Cambridge University Press.
- ↑ IPCC, 2001. Climate Change 2001: The Scientific Basis. Contribution of Working Group I to the Third Assessment Report of the Intergovernmental Panel on Climate Change. J.T. Houghton, Y. Ding, D.J. Griggs, M. Noguer, P.J. van der Linden, X. Dai, K. Maskell and C.A. Johnson (eds.). Intergovernmental Panel on Climate Change. Cambridge University Press, 881pp.
- ↑ Kalnay, E., 2003. Atmospheric Modeling, Data Assimilation and Predictability. Cambridge University Press, 341pp.
- ↑ Majewski, D., D. Liermann, P. Prohl, B. Ritter, M. Buchhold, T. Hanisch, G. Paul, W. Wergen and J. Baumgardner, 2002. The operational global Icosahedral-Hexagonal Gridpoint Model GME: description and high-resolution tests. Monthly Weather Review, 130:319–338.
- ↑ IPCC, 2001. Climate Change 2001: The Scientific Basis. Contribution of Working Group I to the Third Assessment Report of the Intergovernmental Panel on Climate Change. J.T. Houghton, Y. Ding, D.J. Griggs, M. Noguer, P.J. van der Linden, X. Dai, K. Maskell and C.A. Johnson (eds.). Intergovernmental Panel on Climate Change. Cambridge University Press, 881pp.
- ↑ Poulus, G.S., and S.P. Burns, 2003. An evaluation of bulk Ri-based surface layer flux formulas for stable and very stable conditions with intermittent turbulence. Journal of Atmospheric Science, 60:2523–2537.
- ↑ Mahrt, L., 1998: Stratified atmospheric boundary layers and breakdown of models.Theoretical and Computational Fluid Dynamics, 11:263–279.
- ↑ Dethloff, K., C. Abegg, A. Rinke, I. Hebestadt and V.F. Romanov, 2001. Sensitivity of Arctic climate simulations to different boundary-layer parameterizations in a regional climate model.Tellus, 53A:1–26.
- ↑ Randall, D., J. Curry, D. Battisti, G. Flato, R. Grumbine, S. Hakkinen, D. Martinson, R. Preller, J. Walsh and J. Weatherly, 1998. Status of and outlook for large-scale modeling of atmosphere-ice-ocean interactions in the Arctic. Bulletin of the American Meteorological Society, 79:197–219.
- ↑ Takahashi, M., 1999. Simulation of the quasi-biannial oscillation in a general circulation model. Geophysical Research Letters, 26, 1307–1310.
- ↑ Austin, J., D. Shindell, S.R. Beagley, C. Bruhl, M. Damers, E. Manzini, T. Nagashima, P. Newman, S. Pawson, G. Pitari, E. Rozanov, C. Schnadt and T.A. Shepherd, 2003. Uncertainties and assessments of chemistry-climate models of the stratosphere. Atmospheric Chemistry and Physics, 3:1–27.
- ↑ Austin, J., D. Shindell, S.R. Beagley, C. Bruhl, M. Damers, E. Manzini, T. Nagashima, P. Newman, S. Pawson, G. Pitari, E. Rozanov, C. Schnadt and T.A. Shepherd, 2003. Uncertainties and assessments of chemistry-climate models of the stratosphere. Atmospheric Chemistry and Physics, 3:1–27.
- ↑ IPCC, 2001. Climate Change 2001: The Scientific Basis. Contribution of Working Group I to the Third Assessment Report of the Intergovernmental Panel on Climate Change. J.T. Houghton, Y. Ding, D.J. Griggs, M. Noguer, P.J. van der Linden, X. Dai, K. Maskell and C.A. Johnson (eds.). Intergovernmental Panel on Climate Change. Cambridge University Press, 881pp.
- ↑ Bryan, K., S. Manabe and R.C. Pacanowski, 1975. A global ocean-atmosphere climate model. II: the oceanic circulation. Journal of Physical Oceanography, 5:30–46.
- ↑ Randall, D., J. Curry, D. Battisti, G. Flato, R. Grumbine, S. Hakkinen, D. Martinson, R. Preller, J. Walsh and J. Weatherly, 1998. Status of and outlook for large-scale modeling of atmosphere-ice-ocean interactions in the Arctic. Bulletin of the American Meteorological Society, 79:197–219.
- ↑ Sadourny, R., A. Arakawa and Y. Mintz, 1968. Integration of the nondivergent barotropic equation with an icosahedral hexagonal grid on the sphere. Monthly Weather Review, 96:351–356.
- ↑ Murray, R.J., 1996. Explicit generation of orthogonal grids for ocean models. Journal of Computational Physics, 126:251–273.
- ↑ Furevik, T., M. Bentsen, H. Drange, I.K.T. Kindem, N.G. Kvamstø and A. Sorteberg, 2003. Description and validation of the Bergen Climate Model: ARPEGE coupled with MICOM. Climate Dynamics, 21:27–51.
- ↑ Harding, R.J., S.-E. Gryning, S. Halldin and C.R. Lloyd, 2001. Progress in understanding of land surface/atmosphere exchanges at high latitudes. Theoretical and Applied Climatology, 70:5–18.
- ↑ Hagemann, S. and L. Dümenil, 1998. A parameterization of the lateral water flow for the global scale. Climate Dynamics, 14:17–31.
- ↑ Stocker, T.F., G.K.C. Clarke, H. Le Treut, R.S. Lindzen, V.P. Meleshko, R.K. Mugara, T.N. Palmer, R.T. Pierrehumbert, P.J. Sellers, K.E. Trenberth and J. Willebrand, 2001. Physical climate processes and feedbacks. In: J.T. Houghton, Y. Ding, D.J. Griggs, M. Noguer, P.J. van der Linden, X. Dai, K. Maskell and C.A. Johnson (eds.), pp. 418–470. Climate Change 2001: The Scientific Basis. Contribution of Working Group I to the Third Assessment Report of the Intergovernmental Panel on Climate Change. Cambridge University Press.
- ↑ McAvaney, B.J., C. Covey, S. Joussaume, V. Kattsov, A. Kitoh, W. Ogana, A.J. Pitman, A.J. Weaver, R.A. Wood and Z.-C. Zhao, 2001. Model evaluation. In: J.T. Houghton, Y. Ding, D.J. Griggs, M. Noguer, P.J. van der Linden, X. Dai, K. Maskell and C.A. Johnson (eds.). pp. 471–524. Climate Change 2001: The Scientific Basis. Contribution of Working Group I to the Third Assessment Report of the Intergovernmental Panel on Climate Change. Cambridge University Press.
- ↑ IPCC, 2001. Climate Change 2001: The Scientific Basis. Contribution of Working Group I to the Third Assessment Report of the Intergovernmental Panel on Climate Change. J.T. Houghton, Y. Ding, D.J. Griggs, M. Noguer, P.J. van der Linden, X. Dai, K. Maskell and C.A. Johnson (eds.). Intergovernmental Panel on Climate Change. Cambridge University Press, 881pp.
- ↑ Lemke, P., W.D. Hibler III, G.M. Flato, M. Harder and M. Kreyscher, 1997. On the improvement of sea-ice models for climate simulations: The sea-ice model intercomparison project. Annals of Glaciology, 25:183–187.
- ↑ Hunke, E.C. and J.K. Dukowicz, 1997. An elastic-viscous-plastic model for sea ice dynamics. Journal of Physical Oceanography, 27:1849–1867.
- ↑ McAvaney, B.J., C. Covey, S. Joussaume, V. Kattsov, A. Kitoh, W. Ogana, A.J. Pitman, A.J. Weaver, R.A. Wood and Z.-C. Zhao, 2001. Model evaluation. In: J.T. Houghton, Y. Ding, D.J. Griggs, M. Noguer, P.J. van der Linden, X. Dai, K. Maskell and C.A. Johnson (eds.). pp. 471–524. Climate Change 2001: The Scientific Basis. Contribution of Working Group I to the Third Assessment Report of the Intergovernmental Panel on Climate Change. Cambridge University Press.
- ↑ Bitz, C., G. Flato and J. Fyfe, 2002. Sea ice response to wind forcing from AMIP models. Journal of Climate, 15:523–535.–Walsh, J.E., V. Kattsov, W. Chapman, V. Govorkova and T. Pavlova, 2002. Comparison of Arctic climate simulations by uncoupled and coupled global models. Journal of Climate, 15:1429–1446.
- ↑ McAvaney, B.J., C. Covey, S. Joussaume, V. Kattsov, A. Kitoh, W. Ogana, A.J. Pitman, A.J. Weaver, R.A. Wood and Z.-C. Zhao, 2001. Model evaluation. In: J.T. Houghton, Y. Ding, D.J. Griggs, M. Noguer, P.J. van der Linden, X. Dai, K. Maskell and C.A. Johnson (eds.). pp. 471–524. Climate Change 2001: The Scientific Basis. Contribution of Working Group I to the Third Assessment Report of the Intergovernmental Panel on Climate Change. Cambridge University Press.
- ↑ Smith, J.B., M. Hulme, J. Jaagus, S. Keevallik, A. Mekonnen and K. Hailemariam, 1998. Climate change scenarios. In: J.F. Feenstra, I. Burton, J. Smith and R.S.J.Tol (eds.). Handbook on Methods for Climate Change Impact Assessment and Adaptation Strategies, pp. 3-1–3-40.Version 2.0. United Nations Environment Programme and Institute for Environmental Studies, Vrije Universiteit, Amsterdam.
- ↑ Boer, G.J., 2000a. Analysis and verification of model climate. In: P. Mote and A. O’Neill (eds.). Numerical Modeling of the Global Atmosphere in the Climate System. NATO Science Series C-550. Kluwer Academic Publishers.-- Boer, G.J., 2000b. Climate model intercomparison. In: P. Mote and A. O’Neill (eds.). Numerical Modeling of the Global Atmosphere in the Climate System. NATO Science Series C-550. Kluwer Academic Publishers.
- ↑ Gates,W.L., 1992. AMIP:The Atmospheric Model Intercomparison Project. Bulletin of the American Meteorological Society, 73:1962–1970.-- Gates,W.L., J.S. Boyle, C.C. Covey, C.G. Dease, C.M. Doutriaux, R.S. Drach, M. Fiorino, P.J. Gleckler, J.J. Hnilo, S.M. Marlais,T.J. Phillips, G.L. Potter, B.D. Santer, K.R. Sperber, K.E.Taylor and D.N.Williams, 1998. An overview of the results of the Atmospheric Model Intercomparison Project (AMIP). PCMDI Rep. No. 45, UCRL-JC-129928. Program for Climate Model Diagnosis and Intercomparison, Lawrence Livermore National Laboratory, Livermore, CA, 29 pp. + figs.
- ↑ Meehl, G.A., G.J. Boer, C. Covey, M. Latif and R.J. Stouffer, 2000. The Coupled Model Intercomparison Project (CMIP). Bulletin of the American Meteorological Society, 81:313–318.
- ↑ Folland, C., J. Shukla, J. Kinter and M. Rodwell, 2002.The climate of the twentieth century project. Exchanges (Newsletter of CLIVAR), 7(2):37–39.
- ↑ IPCC-TGCIA, 1999. Guidelines on the Use of Scenario Data for Climate Impact and Adaptation Assessment.Version 1. Prepared by T.R. Carter, M. Hulme and M. Lal. Intergovernmental Panel on Climate Change,Task Group on Scenarios for Climate and Impact Assessment, 69pp.
- ↑ Nakicenovic, N. and R. Swart (eds.), 2000. Intergovernmental Panel on Climate Change, Special Report on Emissions Scenarios. Cambridge University Press, 599pp.
- ↑ IPCC, 2001, Op. cit
- ↑ Curry, J.A. and A.H. Lynch, 2002. Comparing Arctic Regional Climate Models. Eos,Transactions, American Geophysical Union, 83:87.;-- see also Section 4.5.1
- ↑ Proshutinsky, A., M. Steele, J. Zhang, G. Holloway, N. Steiner, S. Hakkinen, D. Holland, R. Gerdes, C. Koeberle, M. Karcher, M. Johnson,W. Maslowski,W.Walczowski,W. Hibler and J.Wang, 2001. Multinational effort studies differences among Arctic Ocean models. Eos, Transactions, American Geophysical Union, 82(637):643–644;-- see also Section 4.5.2
- ↑ WCRP, 2002.WOCE/CLIVAR, 2002:WOCE/CLIVAR Working Group on Ocean Model Development. Report of the Third Session. World Climate Research Programme, Informal Rep. No. 14/2002.
- ↑ Braconnot, P. (ed.), 2000. Paleoclimate Modeling Intercomparison Project (PMIP): Proceedings of the Third PMIP Workshop, La Huardière, Canada, 4–8 October 1999. ICPO Publications Series No.34, PAGES 2000–1,WMO/TD No. 1007,WCRP Series Report No. 111, 271pp.
- ↑ Lemke, P.,W.D. Hibler III, G.M. Flato, M. Harder and M. Kreyscher, 1997. On the improvement of sea-ice models for climate simulations: The sea-ice model intercomparison project. Annals of Glaciology, 25:183–187.
- ↑ Etchevers, P., E. Martin, R. Brown, C. Fierz,Y. Lejeune, E. Bazile, A. Boon,Y.-J. Dai, R. Essery, A. Fernandez,Y. Gusev, R. Jordan, V. Koren, E. Kowalczyck, R. Nasonova, D. Pyles, A. Schlosser, A. Shmakin,T.G. Smirnova, U. Strasser, D.Verseghy,T.Yamazakiand Z.-L.Yang, 2002. SnowMIP, an intercomparison of snow models: first results. In: Proceedings of the International Snow Science Workshop, Penticton, Canada, 29 September – 4 October 2002, 8pp.
- ↑ IGPO, 2000. GEWEX Cloud System Study (GCSS) Second Science and Implementation Plan. Global Energy and Water Cycle Experiment (GEWEX). International GEWEX Project Office, Document No. 34, 52pp.
- ↑ Nakiçenoviç, N. and R. Swart (eds.), 2000. Op. cit.
- ↑ Walsh, J.E., V. Kattsov, W. Chapman, V. Govorkova and T. Pavlova, 2002. Comparison of Arctic climate simulations by uncoupled and coupled global models. Journal of Climate, 15:1429–1446.
- ↑ Flato, G.M. and G.J. Boer, 2001. Warming asymmetry in climate change experiments. Geophysical Research Letters, 28:195–198.
- ↑ Boville, B.A., J.T. Kiehl, P.J. Rasch and F.O. Bryan, 2001. Improvements to the NCAR CSM-1 for transient climate simulations. Journal of Climate, 14:164–179.
- ↑ Roeckner, E., J.M. Oberhuber, A. Bacher, M. Christoph and I. Kirchner, 1996. ENSO variability and atmospheric response in a global coupled atmosphere-ocean GCM. Climate Dynamics, 12:737–754.
- ↑ Delworth, T.L., R.J. Stouffer, K.W. Dixon, M.J. Spelman, T.R. Knutson, A.J. Broccoli, P.J. Kushner and R.T. Wetherald, 2002. Simulation of climate variability and change by the GFDL R30 coupled climate model. Climate Dynamics, 19(7):555–574.
- ↑ Gordon, C., C. Cooper, C.A. Senior, H. Banks, J.M. Gregory, T.C. Johns, J.F.B. Mitchell and R.A. Wood, 2000. The simulation of SST, sea ice extents and ocean heat transports in a version of the Hadley Centre coupled model without flux adjustments. Climate Dynamics, 16:147–168.