Introduction to Future Climate Change: Modeling and Scenarios for the Arctic
Published: September 28, 2009, 12:20 pm Updated: May 7, 2012, 4:42 pm Author: International Arctic Science Committee (Introduction to Future Climate Change: Modeling and Scenarios for the Arctic)
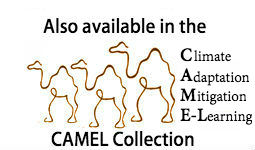
This is Section 4.1 of the Arctic Climate Impact Assessment.
Lead Authors: Vladimir M. Kattsov, Erland Källén; Contributing Authors: Howard Cattle, Jens Christensen, Helge Drange, Inger Hanssen-Bauer,Tómas Jóhannesen, Igor Karol, Jouni Räisänen, Gunilla Svensson, Stanislav Vavulin; Consulting Authors: Deliang Chen, Igor Polyakov, Annette Rinke
To assess climate change impacts on societies, ecosystems, and infrastructure, possible changes in physical climate parameters must first be projected. The physical climate change projections must in turn be calculated from changes in external factors that can affect the physical climate. Examples of such factors include atmospheric composition, particularly atmospheric concentrations of GHGs and aerosols, and land-surface changes (e.g., deforestation). This chapter describes the options available to make such projections and their application to the Arctic. The main emphasis is on physically based models of the climate system and the relationship between global climate change and regional effects in the Arctic.
Physically based climate models are used to obtain climate scenarios – plausible representations of future climate that are consistent with assumptions about future emissions of GHGs and other pollutants (i.e., emissions scenarios) and with present understanding of the effects of increased atmospheric concentrations of these components on the climate[1]. Correspondingly, by using a climate change scenario, the difference between the projected future climate and the current climate is described. Being dependent on sets of prior assumptions about future human activities, demographic and technological change, and their impact on atmospheric composition, climate change scenarios are not predictions, but rather plausible, internally consistent descriptions of possible future climates.
In addition to physical climate modeling, there are alternative methods for providing climate scenarios for use in impact assessments. These include synthetic scenarios (also referred to as arbitrary or incremental scenarios) and analogue scenarios. None of the alternatives provide a physically consistent climate change scenario including both atmospheric composition changes and physically coupled changes in temperature, precipitation, and other climate variables. Nevertheless, due to their relative simplicity they can be useful and adequate for some types of impact studies. There are also climate scenarios that do not fall into any of these categories, which primarily employ extrapolation of either ongoing trends in climate, or future regional climate, on the basis of projected global or hemispheric mean climate change. A separate group of scenarios is based on expert judgments. All of the methods have their limitations, but each has some particular advantages[2].
Synthetic scenarios are based on incremental changes in climatic variables, particularly air temperature (e.g., +1, +2, +3° C) and precipitation (e.g., +5, +10, +15%). Such scenarios often assume a uniform annual change in the variables over the region under consideration; however, some temporal and spatial variability may be introduced as well. Synthetic scenarios provide a framework for conducting sensitivity studies of potential impacts of climate change using impact models. Careful selection of the range and combinations of changes (e.g., using knowledge based on climate model projections), can facilitate "guided" sensitivity analysis, enabling an examination of both the modeled behavior of a system under a plausible range of climatic conditions and the robustness of impact models applied under changed and often unprecedented environmental conditions. Synthetic scenarios can provide a useful context for understanding and evaluating responses to more complex scenarios based on climate model outputs. Transparency to users and limited computational resource requirements, which allow examination of a wide range of potential climate changes (the range is further increased by the possibility of changing individual variables independent of one another), are among the advantages of synthetic scenarios. Their main disadvantage is the lack of internal consistency in applying uniform changes over large and highly variable areas such as the Arctic. Arbitrary changes in different variables may also lead to inconsistencies in synthetic scenarios that can limit their applicability and appropriateness. In addition, synthetic scenarios are not directly related to GHG forcing.
Analogue scenarios of a future climate are of two types: temporal analogue scenarios, which are based on previous warm climate conditions (determined either by instrumental or proxy data), and spatial analogue scenarios, which are based on current climate conditions in warmer regions. The use of historic instrumental records is an apparent advantage of the past climate analogues over other approaches. However, the availability of historic observational data for the Arctic is extremely limited. Proxy climate data, while representing in some cases a physically plausible climate different from the current climate to a degree similar to that of the climate projected for the 21st century, are also not available for many locations. The quality of geological records is often uncertain, and the resolution coarse. Furthermore, the paleoclimate changes are unlikely to have been driven by an increase in GHG concentrations. Spatial analogues are also unrelated to GHG forcing and are often physically implausible. The lack of availability of proper analogues is the major problem for the analogue scenario approach. The IPCC recommends that analogue scenarios are not used, at least not independently of other types of scenario[3].
Physical climate models are based on the laws of physics and discrete numerical representations of these laws that allow computer simulations. Trenberth[4] describes how climate models can be constructed and their underlying physical principles. Of the hierarchy of climate models (Box 4.1), global coupled atmosphere-ocean general circulation models (AOGCMs) are widely acknowledged as the principal, and most promising rapidly developing tools for simulating the response of the global climate system to increasing GHG concentrations. In its Third Assessment Report, the IPCC[5] concluded that state-of-the-art AOGCMs in existence at the turn of the century provided "credible simulations of climate, at least down to subcontinental scales and over temporal scales from seasonal to decadal", and as a class were "suitable tools to provide useful projections of the future climate"[6]. The IPCC[7] identified the following primary sources of uncertainty in climate scenarios based on AOGCM projections: uncertainties in future emissions of GHGs and aerosols (emissions scenarios), and in conversion of the emissions to atmospheric concentrations and to radiative forcing of the climate; uncertainties in the global and regional climate responses to emissions simulated by different AOGCMs; and uncertainties due to inaccurate representation of regional and local climate. A disadvantage of the AOGCMs as a tool for constructing scenarios is their high demand for computational resources, which makes it expensive and time-consuming to carry out calculations for multiple emissions scenarios.
Box 4.1. Climate model hierarchy Climate models have very different levels of complexity with respect to resolution and comprehensiveness. Available computing resources may limit model complexity for practical reasons, but the scientific question to be addressed is the main factor determining the required model complexity. Different levels of reduction (or simplification) create a hierarchy of climate models {{ref|7a} Simple climate models of the energy-balance type, with zero (globally averaged) to two (latitude and height) spatial dimensions, belong to the lowest level of the hierarchy. Based upon parameters derived from more complex climate models, they are useful in studies of climate sensitivity to a particular process over a wide range of parameters (e.g., in a preliminary analysis of climate sensitivity to various emissions scenarios, see Section 4.4.1 (Introduction to Future Climate Change: Modeling and Scenarios for the Arctic)). Simple climate models can also be used as components of integrated assessment models, for example, in analyses of the potential costs of emission reductions or impacts of climate change[8]. Earth system models of intermediate complexity (EMICs) bridge the gap between the simple models and the comprehensive three-dimensional climate models[9].These models explicitly simulate interactions between different components of the climate system; however, at least some of the components have a reduced complexity, potentially limiting their applicability.These models are computationally efficient, allow for long-term climate simulations measured in thousands and tens of thousands of years, and are primarily used for studies of particular climate processes and feedbacks that are not believed to be affected by the dynamical simplifications introduced. Comprehensive three-dimensional coupled atmosphere-ocean general circulation models (AOGCMs) occupy the top level of the hierarchy.The term “general circulation” refers to large-scale flow systems in the atmosphere and oceans, and the associated redistribution of mass and energy in the climate system. General circulation models (GCMs) simulate the behavior of these systems and the interactions between them and with other components of the climate system, such as sea ice, the land surface, and the biosphere. Atmosphere-ocean general circulation models are widely acknowledged as the most sophisticated tool available for global climate simulations, and particularly for projecting future climate states. Atmosphere-ocean general circulation models were preceded by far less computationally demanding atmospheric GCMs coupled to simple parameterizations of the upper mixed layer of the ocean (AGCM/OUML), which still play an important role in studies of processes and feedbacks in the climate system (see also section 4.2.1) and in paleoclimate simulations. While the resolution of AOGCMs used for projections of future climate is rapidly improving, it is still insufficient to capture the fine-scale structure of climatic variables in many regions of the world that is necessary for impact assessment studiesGiorgi, F., B. Hewitson, J. Christensen, M. Hulme, H. von Storch, P.Whetton, R. Jones, L. Mearns and C. Fu, 2001. Regional climate information – evaluation and projections. In: J.T. Houghton, Y. Ding, D.J. Griggs, M. Noguer, P.J. van der Linden, X. Dai, K. Maskell and C.A. Johnson (eds.). pp. 583–638. Climate Change 2001:The Scientific Basis. Contribution of Working Group I to the Third Assessment Report of the Intergovernmental Panel on Climate Change. Cambridge University Press.;-- Mearns et al., 2001, Op. cit.. Hence, a number of techniques exist to enhance the resolution of AOGCM outputs.These techniques fall into three categories: * High- or variable-resolution stand-alone AGCM simulations initialized using atmospheric and land-surface conditions interpolated from the corresponding AOGCM fields and driven with the sea surface temperature and sea-ice distributions projected by the AOGCM. * High-resolution regional (or limited-area) climate models (RCMs) restricted to a domain with simple lateral boundaries, at which they are driven by outputs from GCMs or largerscale RCMs. * Statistical downscaling methods that are based on empirically derived relations between observed large-scale climate variables and local variables, and which apply these relations to the large-scale variables simulated by GCMs (or RCMs). Each of the regionalization techniques is characterized by its own set of advantages and disadvantages. Giorgi et al.Giorgi et al. (2001), Op. cit. provided details on the high- and variable-resolution AGCMs, while RCMs and statistical downscaling are discussed in sections [../155689/index.html 4.5 and 4.6 (Introduction to Future Climate Change: Modeling and Scenarios for the Arctic). |
The selection of climate scenarios for impact assessments is always controversial and vulnerable to criticism[10]. Mearns et al.[11] suggested that, to be useful for impact assessments and policy makers, climate scenarios should be consistent with global projections at the regional level (i.e., projected changes in regional climate may lie outside the range of global mean changes but should be consistent with theory and model-based results); be physically plausible and realistic; provide a sufficient number of variables and appropriate temporal and spatial scales for impact assessments; be representative, reflecting the potential range of future regional climate change; and be accessible.
Compared to the other methods of constructing climate change scenarios, only AOGCMs (possibly in combination with dynamic or statistical downscaling methods) have the potential to provide spatially and physically consistent estimates of regional climate change due to increased atmospheric GHG concentrations[12]. The AOGCM projections are available for a large number of climate variables, at a variety of temporal scales, and for regular grid points all over the world, which should be sufficient for many impact assessments. Employing an ensemble of different models increases the representativeness of AOGCM-based scenarios. When AOGCMs are used to provide the central scenarios, they can be combined with other types of scenarios (e.g., with synthetic scenarios applied at the regional level, for which the AOGCMs provide a physically plausible range of climate changes).
For this assessment, five AOGCMs (referred to as the ACIA-designated models, see Section 4.2.7 (Introduction to Future Climate Change: Modeling and Scenarios for the Arctic)) were selected for constructing future climate change scenarios for the Arctic (see Section 1.4.2 (Introduction to Future Climate Change: Modeling and Scenarios for the Arctic)). The ACIA-designated models are drawn from the generation of climate models evaluated by the IPCC[13]. This chapter begins with a brief description of the state-of-the-art in AOGCM development at the time of the IPCC assessment (Section 4.2 (Introduction to Future Climate Change: Modeling and Scenarios for the Arctic)), followed by an evaluation of the ACIA-designated models’ performance in simulating the current climate of the Arctic (Section 4.3 (Introduction to Future Climate Change: Modeling and Scenarios for the Arctic)). Projections of future climate change in the Arctic using the ACIA-designated models are the central focus of this chapter (Section 4.4 (Introduction to Future Climate Change: Modeling and Scenarios for the Arctic)). An assessment of possible climate change at scales smaller than subcontinental, such as the scale considered by the ACIA (Regional modeling of the Arctic), requires the application of a downscaling technique to the AOGCM output. In this assessment, two methods of downscaling AOGCM projections have been considered: regional climate modeling (Section 4.5 (Introduction to Future Climate Change: Modeling and Scenarios for the Arctic)), and statistical downscaling (Section 4.6 (Introduction to Future Climate Change: Modeling and Scenarios for the Arctic)). Finally, Section 4.7 (Introduction to Future Climate Change: Modeling and Scenarios for the Arctic) presents the outlook for improving AOGCM-based climate change projections for the Arctic.
Chapter 4: Future Climate Change: Modeling and Scenarios for the Arctic
4.1. Introduction
4.2. Global coupled atmosphere-ocean general circulation models
4.3. Simulation of observed arctic climate with the ACIA designated models
4.4. Arctic climate change scenarios for the 21st century projected by the ACIA-designated models
4.5. Regional modeling of the Arctic
4.6. Statistical downscaling approach and downscaling of AOGCM climate change projections
4.7. Outlook for improving climate change projections for the Arctic
References
Citation
Committee, I. (2012). Introduction to Future Climate Change: Modeling and Scenarios for the Arctic. Retrieved from http://editors.eol.org/eoearth/wiki/Introduction_to_Future_Climate_Change:_Modeling_and_Scenarios_for_the_Arctic- ↑ IPCC-TGCIA, 1999. Guidelines on the Use of Scenario Data for Climate Impact and Adaptation Assessment.Version 1. Prepared by T.R. Carter, M. Hulme and M. Lal. Intergovernmental Panel on Climate Change, Task Group on Scenarios for Climate and Impact Assessment, 69pp.
- ↑ Carter, T.R., E.L. La Rovere, R.N. Jones, R. Leemans, L.O. Mearns, N. Nakicenovic, A.B. Pittock, S.M. Semenov and J. Skea, 2001. Developing and applying scenarios. In: J.J. McCarthy, O.F. Canziani, N.A. Leary, D.J. Dokken and K.S. White (eds.). Climate Change 2001: Impacts, Adaptation, and Vulnerability. Pp. 145–190. Contribution of Working Group II to the Third Assessment Report of the Intergovernmental Panel on Climate Change. Cambridge University Press.–Mearns, L.O., M. Hulme, T.R. Carter, R. Leemans, M. Lal and P. Whetton, 2001. Climate scenario development. In: J.T. Houghton, Y. Ding, D.J. Griggs, M. Noguer, P.J. van der Linden, X. Dai, K. Maskell and C.A. Johnson (eds.). pp. 739–768. Climate Change 2001: The Scientific Basis. Contribution of Working Group I to the Third Assessment Report of the Intergovernmental Panel on Climate Change. Cambridge University Press.
- ↑ Carter, T.R., M.L. Parry, H. Harasawa and S. Nishioka, 1994. IPCC Technical Guidelines for Assessing Climate Change Impacts and Adaptations. Department of Geography, University College, London.
- ↑ Trenberth, K. (ed.), 1992. Climate System Modelling. Cambridge University Press, 788pp.
- ↑ IPCC, 2001. Climate Change 2001: The Scientific Basis. Contribution of Working Group I to the Third Assessment Report of the Intergovernmental Panel on Climate Change. J.T. Houghton, Y. Ding, D.J. Griggs, M. Noguer, P.J. van der Linden, X. Dai, K. Maskell and C.A. Johnson (eds.). Intergovernmental Panel on Climate Change. Cambridge University Press, 881pp.
- ↑ McAvaney, B.J., C. Covey, S. Joussaume, V. Kattsov, A. Kitoh, W. Ogana, A.J. Pitman, A.J. Weaver, R.A. Wood and Z.-C. Zhao, 2001. Model evaluation. In: J.T. Houghton, Y. Ding, D.J. Griggs, M. Noguer, P.J. van der Linden, X. Dai, K. Maskell and C.A. Johnson (eds.). pp. 471–524. Climate Change 2001: The Scientific Basis. Contribution of Working Group I to the Third Assessment Report of the Intergovernmental Panel on Climate Change. Cambridge University Press.
- ↑ IPCC, 2001. Climate Change 2001: The Scientific Basis. Contribution of Working Group I to the Third Assessment Report of the Intergovernmental Panel on Climate Change. J.T. Houghton, Y. Ding, D.J. Griggs, M. Noguer, P.J. van der Linden, X. Dai, K. Maskell and C.A. Johnson (eds.). Intergovernmental Panel on Climate Change. Cambridge University Press, 881pp.
- ↑ Mearns, L.O., M. Hulme, T.R. Carter, R. Leemans, M. Lal and P. Whetton, 2001. Climate scenario development. In: J.T. Houghton, Y. Ding, D.J. Griggs, M. Noguer, P.J. van der Linden, X. Dai, K. Maskell and C.A. Johnson (eds.). pp. 739–768. Climate Change 2001: The Scientific Basis. Contribution of Working Group I to the Third Assessment Report of the Intergovernmental Panel on Climate Change. Cambridge University Press.
- ↑ Claussen, M., L.A. Mysak, A.J.Weaver, M. Crucifix,T. Fichefet, M.-F. Loutre, S.L.Weber, J. Alcamo,V.A. Alexeev, A. Berger, R. Calov, A. Ganopolski, H. Goosse, G. Lohmann, F. Lunkeit, I.I. Mokhov,V. Petoukhov, P. Stone and Z.Wang, 2002. Earth system models of intermediate complexity: closing the gap in the spectrum of climate system models. Climate Dynamics, 18:579–586.
- ↑ Smith, J.B., M. Hulme, J. Jaagus, S. Keevallik, A. Mekonnen and K. Hailemariam, 1998. Climate change scenarios. In: J.F. Feenstra, I. Burton, J. Smith and R.S.J. Tol (eds.). Handbook on Methods for Climate Change Impact Assessment and Adaptation Strategies, pp. 3-1–3-40.Version 2.0. United Nations Environment Programme and Institute for Environmental Studies, Vrije Universiteit, Amsterdam.
- ↑ Mearns et al., 2001, Op. cit.
- ↑ IPCC-TGCIA, 1999. Guidelines on the Use of Scenario Data for Climate Impact and Adaptation Assessment.Version 1. Prepared by T.R. Carter, M. Hulme and M. Lal. Intergovernmental Panel on Climate Change, Task Group on Scenarios for Climate and Impact Assessment, 69pp.
- ↑ IPCC, 2001. Climate Change 2001: The Scientific Basis. Contribution of Working Group I to the Third Assessment Report of the Intergovernmental Panel on Climate Change. J.T. Houghton, Y. Ding, D.J. Griggs, M. Noguer, P.J. van der Linden, X. Dai, K. Maskell and C.A. Johnson (eds.). Intergovernmental Panel on Climate Change. Cambridge University Press, 881pp.
- ↑ McAvaney et al., 2001, Op. cit.