Statistical downscaling approach and downscaling of AOGCM climate change projections
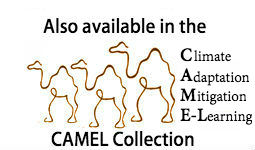
This is Section 4.6 of the Arctic Climate Impact Assessment.
Lead Authors: Vladimir M. Kattsov, Erland Källén; Contributing Authors: Howard Cattle, Jens Christensen, Helge Drange, Inger Hanssen-Bauer,Tómas Jóhannesen, Igor Karol, Jouni Räisänen, Gunilla Svensson, Stanislav Vavulin; Consulting Authors: Deliang Chen, Igor Polyakov, Annette Rinke
Statistical downscaling (also called empirical downscaling) is a tool for downscaling climate information from coarse spatial scales to finer scales. It may be applied as an alternative, or as a supplement, to dynamic downscaling (i.e., regional modeling).The underlying concept is that local climate is conditioned by large-scale climate and by local physiographical features such as topography, distance to a coast, and vegetation[1]. At a specific location, therefore, links should exist between large-scale and local climatic conditions. Statistical downscaling consists of identifying empirical links between large-scale patterns of climate elements (predictors) and local climate (the predictand), and applying them to output from global or regional models. Successful statistical downscaling is thus dependent on long reliable series of predictors and predictands. Giorgi et al. (2001)[2] provide a survey of statistical downscaling studies with emphasis on studies published between 1995 and 2000.
Contents
Approach (4.6.1)
Predictands (4.6.1.1)
Although mean temperature and precipitation (seasonal, monthly, or daily) are the most commonly used local predictands, statistical downscaling has also been applied to generate local scenarios of cloud cover, daily temperature range, extreme temperatures, relative humidity, sunshine (Sunlight) duration, snow-cover duration, and sea-level anomalies.[3] Even sea ice,[4] ocean salinity and oxygen concentrations,[5] zooplankton,[6] and phytoplankton spring blooms in a Swedish lake[7] have been used as predictands.
Predictors (4.6.1.2)
The large-scale predictors should satisfy certain conditions: they should be reproduced realistically by the particular global model; they should (alone or combined) be able to account for most of the observed variations in the predictand; the statistical relationships should be physically interpretable and temporally stationary; and, when applied to a changing climate, predictors that “carry the climate change signal” should be included.[8]
The optimal choice of predictors depends upon the predictand. For downscaling local temperature, large-scale fields of geopotential height or air temperature might be used as the “signal-carrying” predictors (e.g., Huth, 1999).[9] For precipitation, absolute or specific humidity may be used.[10] In maritime regions, air temperature can sometimes serve as a proxy for humidity.[11] In addition, some indicator of atmospheric circulation (e.g., sea-level pressure or a geopotential height field) is usually included (e.g., Chen and Chen, 2003)[12]
Methods (4.6.1.3)
Surveys of methods for establishing links between largescale predictors and local predictands are provided by Hewitson and Crane (1996), Zorita and von Storch (1997), Wilby and Wigley (1997), Xu (1999), Giorgi et al. (2001), and Mearns et al. (2001).[13] The choice of method should depend on predictand, time resolution, and also on the application of the scenario. Linear methods such as canonical correlation analysis (CCA), singular value decomposition (SVD), and multiple linear regression analysis (MLR) can, in most cases, be used to generate scenarios of monthly or seasonal values (e.g., Busuioc et al., 1999; Corte-Real et al., 1995; Huth and Kysely, 2000; Sailor and Li, 1999).[14] To generate scenarios for variables such as daily precipitation, however, nonlinear techniques such as weather classification (e.g., Conway and Jones, 1998; Enke and Spekat, 1997; Goodess and Palutikof, 1998, Palutikof et al., 2002; Schnur and Lettenmaier, 1997),[15] neural nets,[16] or analogues[17] are most useful.Weather generators (e.g., Semenov and Barrow, 1997; Semenov et al., 1998; Wilby et al., 1998; Wilks, 1999)[18] can also be applied for generating scenarios with daily resolution, starting from monthly climate-change scenarios generated by one of the above methods.
Comparison of statistical downscaling and regional modeling (4.6.1.4)
Several studies have compared results from statistical and regional modeling.[19] The main impression from these studies is that results from the two downscaling techniques are usually quite similar for present-day climate, while differences in future climate projections are found more frequently. These differences can, to a large degree, be explained by the unwise choice of predictors in the statistical downscaling, for example, predictors that carry the climate signal.[20] It has also been suggested that results from statistical downscaling may be misleading because the projected climate change exceeds the range of data used to develop the model.[21] However, differences between results from statistical downscaling and regional modeling may also result from the ability of statistical downscaling to reproduce local features that are not resolved in the regional models.[22]
Some disadvantages of statistical downscaling versus regional modeling are as follows.
- The major weakness of statistical downscaling is the assumption that observed links between large-scale predictors and local predictands will persist in a changed climate.
- A problem when applying statistical downscaling techniques to daily values is that the observed autocorrelation between the weather at consecutive time steps is not necessarily reproduced. It it is essential to reproduce this, a sutiable method (e.g., weather generators; Katz and Parlange, 1996; Wilks, 1999)[23] should be used.
- Statistical downscaling does not necesssarilty reproduce a physically sound relationship between different climate elements. Using a downscaling method based on weather classification for several predictands (e.g., Enke and Spekat, 1997)[23] can minimize this problem.
- Successful statistical downscaling depends on long, reliable observational series of predictors and predictands.
Some advantages of statistical downscaling versus regional modeling are as follows:
- Statistical downscaling is less technically demanding than regional modeling. It is thus possible to downscale from several GCMs and several different emissions scenarios relatively quickly and inexpensively.[24]
- It is possible to tailor scenarios for specific localities, scales, and problems. The spatial resolution applied in regional climate modeling is still too coarse for many impact studies, and some variables are either not available or not realistically reproduced by regional models. For example, Omstedt and Chen (2001)[25] applied statistical downscaling to infer sea-ice extent in the Baltic Sea.
- In most cases, the development of statistical downscaling models includes an evaluation of AOGCM performance in simulating the climate of a specific region.[26] Methods applied in statistical downscaling have been used to evaluate large-scale fields of single variables as well as the links between different fields.[27] The stability of these links under global change has also been investigated (e.g., Chen and Hellstrom, 1999).[28] Such analyses can indicate which variables serve as the best predictors.
Statistical downscaling of AOGCM climate change projections in the Arctic (4.6.2)
A survey of statistical downscaling studies up to 2000 is provided by the IPCC.[29] However, very few of these studies considered arctic sites. During the 1990s, a few statistical downscaling studies in the Atlantic/European sector of the Arctic were performed at the Danish Meteorological Institute. Kaas and Frich (1995)[30] downscaled monthly means of diurnal temperature range (DTR) and cloud cover at ten synoptic stations from Greenland in the west to Finland in the east. The 500 hPa height and the 500/1000 hPa thickness anomaly fields were used as predictors in an MLR-based model.The model predictors were taken from the final 30 years of a control simulation of the 20th century and the final 30 years of a scenario “A” (business as usual) simulation of the 21st century generated by the ECHAM1 model.[31] Kaas and Frich (1995) found that statistically significant negative trends in DTR were projected for Fennoscandia, especially in central and eastern areas, and especially during winter. For Greenland and Iceland, only minor trends in DTR were projected. Positive trends in cloud cover were projected over most of the area; these were most significant in northeastern areas of Fennoscandia.
In Canada, artificial neural networks (ANNs) have been applied to model hydrological variables.[32] Clair et al. (1998)[33] present a scenario for changed runoff from different Canadian ecozones (including arctic areas) under conditions of doubled atmospheric CO2 concentrations. A doubled-CO2 equilibrium climate change scenario produced by the Canadian climate model CCC[34] was used to generate scenarios for individual basins. Temperature and precipitation scenarios were fed into the ANN to produce scenarios of changes in runoff. In the arctic ecozones that were investigated in the study, the projected changes in annual runoff were between 0 and +10%, the spring melt advanced by between a couple of weeks and one month, and there was a tendency for reduced runoff during summer. Qualitatively similar findings are reported in section 6.8, where annual discharge from various North American rivers to the Arctic Ocean is projected to increase by 10 to 25% during the 21st century.
Recently, most of the statistical downscaling studies for the Arctic have been performed for the European sector as part of the Norwegian Regional Climate Development Under Global Warming (RegClim) project or the Swedish Regional Climate Modelling Programme (SWECLIM), both of which use regional modeling and statistical downscaling to generate climate scenarios. Statistical downscaling using results from several global models and for various emissions scenarios has been completed.[35] The primary global model used in the RegClim project was ECHAM4/OPYC3,[36] forced with the IS92a emissions scenario. The primary case studied was GSDIO, which included changes in GHGs, tropospheric ozone, and direct as well as indirect sulfur aerosol forcing.[37]
In SWECLIM, the main global models were HadCM2 [38] and ECHAM4/OPYC3. Hanssen-Bauer and Førland (2001)[39] evaluated the ECHAM4/OPYC3 simulation of present-day climate over Norway and Svalbard, while Räisänen and Döscher (1999)[40] evaluated the HadCM2 simulation of the present-day climate of northern Europe. Sea-level pressure has proven to be a good indicator for the Scandinavian climate,[41] and was therefore used as a large-scale predictor in the statistical downscaling models. Depending on predictands, additional predictors included air temperature at 2 m height, humidity, and precipitation. The models were developed by linear techniques: CCA, SVD, or MLR. Monthly precipitation and temperatures at selected stations were the main predictands.[42] Although not used for scenario estimation, some non-standard climate variables such as annual maximum sea ice extent over the Baltic Sea,[43] sea level near Stockholm,[44] and spring phytoplankton blooms in a Swedish lake[45] were also linked to atmospheric circulation and may thus be projected by statistical downscaling.
The statistically downscaled temperature scenario based upon the GSDIO integration projects increases in mean annual temperature of 0.2 to 0.5ºC per decade in Norway up to 2050,[46] and 0.6ºC per decade in Svalbard.[47] Cumulative frequency (relative number of years that
temperatures go below a certain threshold given as a function of the threshold value) plots of annual and seasonal mean temperatures based on this study (Fig. 1) show good correspondence between observations and model output for time slices within the 20th century. The smallest warming rates were simulated in southern Norway along the coast; the rates increase when moving inland and northward. Along the coast of southern Norway, the modeled warming rates are similar in all seasons. Farther north and in the interior, considerably larger warming rates are projected for winter than for summer. Comparing these results to the results from dynamic downscaling of the same integration[48] shows only minor differences in summer and autumn. In winter and spring, on the other hand, the statistical downscaling projects greater warming rates for locations that are exposed to temperature inversions. Hanssen-Bauer et al. (2003)[49] argue that it is reasonable to expect weaker winter inversions in the future, and that greater winter warming rates should be expected in valleys compared to mountains.
Benestad (2002, 2004)[50] shows that statistical downscaling from several different climate models gives different local warming rates over Fennoscandia. Still, the temperature signal is robust in some respects: all models simulate warming; the warming is larger inland than along the coast; and the seasonal patterns are similar. A comparison of statistically downscaled temperature scenarios for Svalbard based on the GSDIO integration and HadCM3, both forced with the IS92a emissions scenario, and NCAR’s CSM forced with a 1% per year increase in atmospheric CO2 concentration, revealed differences (Fig. 2) that to a large degree can be explained in terms of different descriptions of sea-ice extent.[51] The HadCM3 model, which projects significantly stronger warming in this area than the other simulations, projects a substantial retreat of sea ice in the Barents Sea.
The CSM model, which projects the most moderate warming rates, shows no melting of sea ice this area. Local temperature scenarios in the high Arctic are thus closely related to the projected changes in regional sea-ice cover. If the AOGCM fails to reproduce either the present sea-ice border or future melting in the region, the local temperature projections will be suspect. Hellström et al. (2001)[52] compared two dynamically and statistically downscaled precipitation scenarios for Sweden.The precipitation climates of the GCMs, dynamic models (i.e., RCMs), and statistical models from the control runs were also compared with respect to their ability to reproduce the observed seasonal cycle (Fig. 3). Improvements in the representation of the seasonal cycle by the downscaling models compared to the GCMs significantly increase the credibility of the downscaling models.
Chen et al. (2001)[53] applied statistically downscaled scenarios from 17 CMIP2 AOGCMs to quantify AOGCM-related uncertainty in the estimation of precipitation scenarios.The result shows that there is an overall projected increase in annual precipitation over the 21st century throughout Sweden.The projected increase is greater in northern than southern Sweden.The precipitation in autumn, winter, and spring is projected to increase throughout the country, whereas decreasing summer precipitation is projected for the southern part of the country.The estimates for winter have a higher level of confidence than the estimates for summer. A statistically downscaled precipitation scenario based upon the GSDIO integration[54] also projects increased annual precipitation in Norway. The projected rates of increase are smallest in southeastern Norway, where they are not statistically significant, and greatest along the northwestern and western coast, where they are highly significant (Fig. 4). In winter and autumn, statistically significant positive trends are projected for most of Norway, while most of the modeled changes in spring and summer precipitation are not statistically significant.
To date, statistical downscaling has primarily concentrated on monthly and annual scales. However, Linderson et al. (2004)[55] developed downscaling models for daily statistics based upon monthly precipitation values for southern Sweden. Future scenarios of selected daily precipitation statistics were downscaled from a GCM developed at the Canadian Centre for Climate Modelling and Analysis (CGCM1; e.g., Flato et al., 2000).[56] The downscaling models use large-scale precipitation, relative humidity, and circulation indices as predictors.The models are skillful in reproducing the variability of mean precipitation and the frequency of days with no precipitation, but less skillful concerning extremes and statistics of days with precipitation. By the time that atmospheric CO2 (Anthropogenic carbon dioxide emissions) doubles in the model, the CGCM1 projects an increase of 10% in annual mean precipitation (statistically significant at the 95% level), and an insignificant reduction in the annual frequency of days with precipitation of 1.4%. An increase in precipitation intensity is projected throughout most of the year, especially during winter. The major changes include a substantial increase in winter precipitation, a delay in the timing of the summer maximum, and prolonged duration of the winter maximum relative to present-day climate.This indicates a more maritime precipitation climate in the scenario climate compared to the control climate. Statistically downscaled climate scenarios for the North American and Russian parts of the Arctic have not yet been published. However, the Canadian Climate Impacts Scenarios (CCIS) Project has validated a downscaling tool, the Statistical DownScaling Model (SDSM).[57] The CCIS Project is providing predictor variable data to support the SDSM.
Summary (4.6.3)
Although only a few statistical downscaling studies have been performed for arctic localities so far, results from the available studies indicate that these methods are able to resolve projected changes in temperature gradients between the coast and inland, and changes in valley temperature inversions.They also generate a more realistic representation of the annual precipitation cycle than the AOGCMs. If careful attention is given to the choice of predictors, scenarios from statistical downscaling and regional modeling seem to be consistent. However, statistical downscaling does not necessarily reproduce a physically based relationship between different climate elements. Conversely, the ability to downscale from several models and integrations is useful for assessing uncertainty. Results from downscaling temperature scenarios from several models underscore that the local temperature projections in the high Arctic depend on how well changes in sea-ice extent are represented in the AOGCMs.
Chapter 4: Future Climate Change: Modeling and Scenarios for the Arctic
4.1. Introduction (Statistical downscaling approach and downscaling of AOGCM climate change projections)
4.2. Global coupled atmosphere-ocean general circulation models
4.3. Simulation of observed arctic climate with the ACIA designated models
4.4. Arctic climate change scenarios for the 21st century projected by the ACIA-designated models
4.5. Regional modeling of the Arctic
4.6. Statistical downscaling approach and downscaling of AOGCM climate change projections
4.7. Outlook for improving climate change projections for the Arctic
References
- Kaas, E. and P. Frich, 1995. Diurnal temperature range and cloud cover in the Nordic countries: observed trends and estimates for the future. Atmospheric Research, 37:211–228.
- Martin, E., B.Timbal and E. Brun, 1997. Downscaling of general circulation model output: simulation of snow climatology of the French Alps and sensitivity to climate change. Climate Dynamics, 13:45–56.
- Schubert, S., 1998. Downscaling local extreme temperature changes in south-eastern Australia from the CSIRO MARK2 GCM. International Journal of Climatology, 18:1419–1439.
- Solman, S.A. and M.N. Nuñez, 1999. Local estimates of global climate change: A statistical downscaling approach. International Journal of Climatology, 19:835–861.
- Wilby, R.L. and T.M.L.Wigley, 2000. Precipitation predictors for downscaling: observed and general circulation model relationships. International Journal of Climatology, 20:641–661.
- Wilby, R.L. and T.M.L.Wigley, 1997. Downscaling general circulation model output: a review of methods and limitations. Progress in Physical Geography, 21:530–548.
- Xu 1999
- Giorgi, F., B. Hewitson, J. Christensen, M. Hulme, H. von Storch, P.Whetton, R. Jones, L. Mearns and C. Fu, 2001. Regional climate information – evaluation and projections. In: J.T. Houghton, Y. Ding, D.J. Griggs, M. Noguer, P.J. van der Linden, X. Dai, K. Maskell and C.A. Johnson (eds.). pp. 583–638. Climate Change 2001:The Scientific Basis. Contribution of Working Group I to the Third Assessment Report of the Intergovernmental Panel on Climate Change. Cambridge University Press.
- Mearns, L.O., M. Hulme,T.R. Carter, R. Leemans, M. Lal and P.Whetton, 2001. Climate scenario development. In: J.T. Houghton,Y. Ding, D.J. Griggs, M. Noguer, P.J. van der Linden, X. Dai, K. Maskell and C.A. Johnson (eds.). pp. 739–768. Climate Change 2001:The Scientific Basis. Contribution of Working Group I to the Third Assessment Report of the Intergovernmental Panel on Climate Change. Cambridge University Press.
- Huth, R. and J. Kysely, 2000. Constructing site-specific climate change scenarios on a monthly scale using statistical downscaling. Theoretical and Applied Climatology, 66:13–27.
- Sailor, D.J. and X. Li, 1999. A semiempirical downscaling approach for predicting regional temperature impacts associated with climatic change. Journal of Climate, 12:103–114.
- Goodess, C.M. and J.P. Palutikof, 1998. Development of daily rainfall scenarios for southeast Spain using a circulation-type approach to downscaling. International Journal of Climatology, 10:1051–1083.
- Palutikof, J.P., C.M. Goodess, S.J. Watkins and T. Holt, 2002. Generating daily rainfall and temperature scenarios at multiple sites: Examples from the Mediterranean. Journal of Climate, 15:3529–3548.
- Schnur, R. and D. Lettenmaier, 1997. A case study of statistical downscaling in Australia using weather classification by recursive partitioning. Journal of Hydrology, 211:362–379.
- Crane, R.G. and B.C. Hewitson, 1998. Doubled CO2 precipitation changes for the Susquehanna Basin: downscaling from the genesis General Circulation Model. International Journal of Climatology, 18:65–76.
- Schoof, J.T. and S.C. Pryor, 2001. Downscaling temperature and precipitation: A comparison of regression-based methods and artificial neural networks. International Journal of Climatology, 21:773–790.
- Wilby, R.L.,T.M.L.Wigley, D. Conway, P.D. Jones, B.C. Hewitson, J. Main and D.S.Wilks, 1998. Statistical downscaling of general circulation model output: A comparison of methods.Water Resources Research, 34:2995–3008.
- Wilks, D., 1999. Multisite downscaling of daily precipitation with a stochastic weather generator. Climate Research, 11:125–136.
- Kidson, J.W. and C.S.Thompson, 1998. Comparison of statistical and model-based downscaling techniques for estimating local climate variations. Journal of Climate, 11:735–753.
- Murphy, J., 1999. An evaluation of statistical and dynamical techniques for downscaling local climate. Journal of Climate, 12:2256–2284.
- Murphy, J., 2000. Predictions of climate change over Europe using statistical and dynamical downscaling techniques. International Journal of Climatology, 20:489–501.
- ^ Katz, R.W. and M.B. Parlange, 1996. Mixtures of stochastic processes: applications to statistical downscaling. Climate Research, 7:185–193.
- Wilks, D., 1999. Multisite downscaling of daily precipitation with a stochastic weather generator. Climate Research, 11:125–136.
- Wilby, R.L. and T.M.L.Wigley, 2000. Precipitation predictors for downscaling: observed and general circulation model relationships. International Journal of Climatology, 20:641–661.
- Ehrman, J., K. Higuchi and T.A. Clair, 2000. Backcasting to test the use of neural networks for predicting runoff in Canadian rivers. Canadian Water Resources Journal, 25(3):279–291.
- Chen, D., C. Achberger, J. Räisänen and C. Hellström, 2001. Using statistical downscaling to quantify the GCM-related uncertainty in regional climate change scenarios: A case study of Swedish precipitation. SWECLIM Newsletter, Swedish Meteorological and Hydrological Institute, Norrköping, Sweden.
- Chen, D. and C. Hellström, 1999.The influence of the North Atlantic Oscillation on the regional temperature variability in Sweden: spatial and temporal variations.Tellus, 51A(4):505–516.
- Hanssen-Bauer, I. and E.J. Førland, 2001.Verification and analysis of a climate simulation of temperature and pressure fields over Norway and Svalbard. Climate Research, 16:225–235.
- Busuioc, A., D. Chen and C. Hellström, 2001a. Performance of statistical downscaling models in GCM validation and regional climate estimates: application for Swedish precipitation. International Journal of Climatology, 21:557–578.
- Chen, D. and Y. Chen, 1999. Development and verification of a multipleregression downscaling model for monthly temperature in Sweden. In: D. Chen, C. Hellström,Y. Chen (eds.). Preliminary Analysis and Statistical Downscaling of Monthly Temperature in Sweden, pp. 41–55. Report C16, Earth Sciences Centre, University of Gothenburg, Sweden.
- Hanssen-Bauer, I., E.J. Førland, J.E. Haugen and O.E.Tveito, 2003. Temperature and precipitation scenarios for Norway: Comparison of results from dynamical and empirical downscaling. Climate Research, 25:15–27.
- Hellström, C., D. Chen, C. Achberger and J. Räisänen, 2001. Comparison of climate change scenarios for Sweden based on statistical and dynamical downscaling of monthly precipitation. Climate Research, 19:45–55.
Citation
Committee, I. (2012). Statistical downscaling approach and downscaling of AOGCM climate change projections. Retrieved from http://editors.eol.org/eoearth/wiki/Statistical_downscaling_approach_and_downscaling_of_AOGCM_climate_change_projections- ↑ von Storch,H.,1999.The global and regional climate system. In:H.von Storch and G.Flöser (eds.).Anthropogenic Climate Change,pp.3–36.Springer Verlag.
- ↑ Giorgi, F., B. Hewitson, J. Christensen, M. Hulme, H. von Storch, P.Whetton, R. Jones, L. Mearns and C. Fu, 2001. Regional climate information – evaluation and projections. In: J.T. Houghton, Y. Ding, D.J. Griggs, M. Noguer, P.J. van der Linden, X. Dai, K. Maskell and C.A. Johnson (eds.). pp. 583–638. Climate Change 2001:The Scientific Basis. Contribution of Working Group I to the Third Assessment Report of the Intergovernmental Panel on Climate Change. Cambridge University Press.
- ↑ Enke,W. and A. Spekat, 1997. Downscaling climate model outputs into local and regional weather elements by classification and regression. Climate Research, 8:195–207.Heyden, H., E. Zorita and H.Von Storch, 1996. Statistical downscaling of monthly mean North Atlantic air-pressure to sea level anomalies in the Baltic Sea.Tellus, 48A:312–323
- ↑ Omstedt, A. and D. Chen, 2001. Influence of atmospheric circulation on the maximum ice extent in the Baltic Sea. Journal of Geophysical Research, 106(C3):4493–4500.
- ↑ (Zorita and Laine, 2000)
- ↑ Heyden, H., H. Fock and W. Greve, 1998. Detecting relationships between interannual variability in ecological time series and climate using a multivariate statistical approach – a case study on Helgoland Road zooplankton. Climate Research, 10:179–191.
- ↑ Blenckner,T. and D. Chen, 2003. Comparison of the impact of regional and north-Atlantic atmospheric circulation on an aquatic ecosystem. Climatic Research, 23:131–136.
- ↑ Giorgi, F., B. Hewitson, J. Christensen, M. Hulme, H. von Storch, P.Whetton, R. Jones, L. Mearns and C. Fu, 2001. Regional climate information – evaluation and projections. In: J.T. Houghton, Y. Ding, D.J. Griggs, M. Noguer, P.J. van der Linden, X. Dai, K. Maskell and C.A. Johnson (eds.). pp. 583–638. Climate Change 2001:The Scientific Basis. Contribution of Working Group I to the Third Assessment Report of the Intergovernmental Panel on Climate Change. Cambridge University Press.
- ↑ Huth, R., 1999. Statistical downscaling in central Europe: Evaluation of methods and potential predictors. Climate Research, 13:91–101.
- ↑ Crane, R.G. and B.C. Hewitson, 1998. Doubled CO2 precipitation changes for the Susquehanna Basin: downscaling from the genesis General Circulation Model. International Journal of Climatology,18:65–76.Hellström, C., D. Chen, C. Achberger and J. Räisänen, 2001. Comparison of climate change scenarios for Sweden based on statistical and dynamical downscaling of monthly precipitation. Climate Research, 19:45–55.
- ↑ Wilby, R.L. and T.M.L.Wigley, 2000. Precipitation predictors for downscaling: observed and general circulation model relationships. International Journal of Climatology, 20:641–661.
- ↑ Chen, D., and Y. Chen, 2003. Association between winter temperature in China and upper air circulation over East Asia revealed by Canonical Correlation Analysis. Global and Planetary Change, 37:315–325.
- ↑ Hewitson, B.C. and R.G. Crane, 1996. Climate downscaling: techniques and application. Climate Research, 7:85–95.Zorita and von Storch (1997)
- ↑ : Busuioc, A., H. von Storch and R. Schnur, 1999.Verification of GCM-generated regional seasonal precipitation for current climate and of statistical downscaling estimates under changing climate conditions. Journal of Climate, 12:258–272.Corte-Real, J., X. Zhang and X.Wang, 1995. Large-scale circulation regimes and surface climate anomalies over the Mediterranean. International Journal of Climatology, 15:1135–1150.
- ↑ Conway, D. and P.D. Jones, 1998.The use of weather types and air flow indices for GCM downscaling. Journal of Hydrology, 212:348–361.Enke,W. and A. Spekat, 1997. Downscaling climate model outputs into local and regional weather elements by classification and regression. Climate Research, 8:195–207.
- ↑ Cavazos,T., 1999: Large-scale circulation anomalies conductive to extreme precipitation events and derivation of daily rainfall in northeastern Mexico and southeastern Texas. Journal of Climate, 12:1506–1523.Clair,T.A. and J. Ehrman, 1998. Using neural networks to assess the influence of changing seasonal climates in modifying discharge, dissolved organic carbon, and nitrogen export in eastern Canadian rivers.Water Resources Research, 34(3):447–455.
- ↑ (Zorita and von Storch, 1999)
- ↑ Semenov, M.A. and E. Barrow, 1997. Use of stochastic weather generator in the development of climate change scenarios. Climatic Change, 35:397–414.Semenov, M.A., R.J. Brooks, E.M. Barrow and C.W. Richardson, 1998. Comparison of the WGEN and LARS-WG stochastic weather generators for diverse climates. Climate Research, 10:95–107.
- ↑ Cubasch, U., H. von Storch, J.Waszkewitz and E. Zorita, 1996. Estimates of climate change on southern Europe derived from dynamical climate model output. Climate Research, 7:129–149.Hellström, C., D. Chen, C. Achberger and J. Räisänen, 2001. Comparison of climate change scenarios for Sweden based on statistical and dynamical downscaling of monthly precipitation. Climate Research, 19:45–55.
- ↑ Murphy, J., 2000. Predictions of climate change over Europe using statistical and dynamical downscaling techniques. International Journal of Climatology, 20:489–501.
- ↑ Mearns, L.O., M. Hulme,T.R. Carter, R. Leemans, M. Lal and P.Whetton, 2001. Climate scenario development. In: J.T. Houghton,Y. Ding, D.J. Griggs, M. Noguer, P.J. van der Linden, X. Dai, K. Maskell and C.A. Johnson (eds.). pp. 739–768. Climate Change 2001:The Scientific Basis. Contribution of Working Group I to the Third Assessment Report of the Intergovernmental Panel on Climate Change. Cambridge University Press.
- ↑ Hanssen-Bauer, I., E.J. Førland, J.E. Haugen and O.E.Tveito, 2003. Temperature and precipitation scenarios for Norway: Comparison of results from dynamical and empirical downscaling. Climate Research, 25:15–27.
- ↑ Enke,W. and A. Spekat, 1997. Downscaling climate model outputs into local and regional weather elements by classification and regression. Climate Research, 8:195–207.
- ↑ Benestad, R., 2002. Empirically downscaled multi-model ensemble temperature and precipitation scenarios for Norway. Journal of Climate, 15:3008–3027.
- ↑ Omstedt, A. and D. Chen, 2001. Influence of atmospheric circulation on the maximum ice extent in the Baltic Sea. Journal of Geophysical Research, 106(C3):4493–4500.
- ↑ Busuioc, A., H. von Storch and R. Schnur, 1999.Verification of GCM-generated regional seasonal precipitation for current climate and of statistical downscaling estimates under changing climate conditions. Journal of Climate, 12:258–272.Busuioc, A., D. Chen and C. Hellström, 2001a. Performance of statistical downscaling models in GCM validation and regional climate estimates: application for Swedish precipitation. International Journal of Climatology, 21:557–578.
- ↑ Busuioc, A., D. Chen and C. Hellström, 2001b.Temporal and spatial variability of precipitation in Sweden and its link with the large scale atmospheric circulation.Tellus, 53A(3):348–367.Hanssen-Bauer, I. and E.J. Førland, 2001.Verification and analysis of a climate simulation of temperature and pressure fields over Norway and Svalbard. Climate Research, 16:225–235.
- ↑ Chen, D. and C. Hellström, 1999.The influence of the North Atlantic Oscillation on the regional temperature variability in Sweden: spatial and temporal variations.Tellus, 51A(4):505–516.
- ↑ Giorgi, F., B. Hewitson, J. Christensen, M. Hulme, H. von Storch, P.Whetton, R. Jones, L. Mearns and C. Fu, 2001. Regional climate information – evaluation and projections. In: J.T. Houghton, Y. Ding, D.J. Griggs, M. Noguer, P.J. van der Linden, X. Dai, K. Maskell and C.A. Johnson (eds.). pp. 583–638. Climate Change 2001:The Scientific Basis. Contribution of Working Group I to the Third Assessment Report of the Intergovernmental Panel on Climate Change. Cambridge University Press.
- ↑ Kaas, E. and P. Frich, 1995. Diurnal temperature range and cloud cover in the Nordic countries: observed trends and estimates for the future. Atmospheric Research, 37:211–228.
- ↑ Cubasch, U., K. Hasselmann, H. Höck, E. Maier-Raimer, U. Mikolajewicz, B.D. Santer and R. Sausen, 1992.Time-dependent greenhouse warming computations with a coupled oceanatmosphere model. Climate Dynamics, 9:55–69.
- ↑ Clair,T.A. and J. Ehrman, 1998. Using neural networks to assess the influence of changing seasonal climates in modifying discharge, dissolved organic carbon, and nitrogen export in eastern Canadian rivers.Water Resources Research, 34(3):447–455.Clair,T.A., J. Ehrman and K. Higuchi, 1998. Changes to the runoff of Canadian ecozones under a doubled CO2 atmosphere. Canadian Journal of Fisheries and Aquatic Sciences, 55(11):2464–2477.
- ↑ Clair,T.A., J. Ehrman and K. Higuchi, 1998. Changes to the runoff of Canadian ecozones under a doubled CO2 atmosphere. Canadian Journal of Fisheries and Aquatic Sciences, 55(11):2464–2477.
- ↑ Boer, G.J., N.A. McFarlane and M. Lazare, 1992. Greenhouse gasinduced climate change simulated with the CCC secondgeneration general circulation model. Journal of Climate, 5:1045–1077.
- ↑ Benestad, R., 2002. Empirically downscaled multi-model ensemble temperature and precipitation scenarios for Norway. Journal of Climate, 15:3008–3027.Benestad, R., 2004.Tentative probabilistic temperature scenarios for northern Europe.Tellus A: Dynamic Meteorology and Oceanography, 56:89–101.
- ↑ Roeckner, E., J.M. Oberhuber, A. Bacher, M. Christoph and I. Kirchner, 1996. ENSO variability and atmospheric response in a global coupled atmosphere-ocean GCM. Climate Dynamics, 12:737–754.Roeckner, E., L. Bengtsson, J. Feichter, J. Lelieveld and H. Rodhe,1999.Transient climate change simulations with a coupled atmosphere-ocean GCM including the tropospheric sulfur cycle. Journal of Climate, 12:3004–3032.
- ↑ Roeckner, E., L. Bengtsson, J. Feichter, J. Lelieveld and H. Rodhe,1999.Transient climate change simulations with a coupled atmosphere-ocean GCM including the tropospheric sulfur cycle. Journal of Climate, 12:3004–3032.
- ↑ Johns,T.C., 1996. A description of the second Hadley Centre coupled model (HADCM2). Report No. 71. Hadley Centre for Climate Prediction and Research, UK.Johns,T.C., R.E. Carnell, J.F. Crossley, J.M. Gregory, J.F.B. Mitchell, C.A. Senior, S.F.B.Tett and R.A.Wood, 1997.The second Hadley Centre coupled atmosphere-ocean GCM: model description, spinup and validation. Climate Dynamics, 13:103–134.
- ↑ Hanssen-Bauer, I. and E.J. Førland, 2001.Verification and analysis of a climate simulation of temperature and pressure fields over Norway and Svalbard. Climate Research, 16:225–235.
- ↑ Räisänen and Döscher (1999)
- ↑ Busuioc, A., D. Chen and C. Hellström, 2001b.Temporal and spatial variability of precipitation in Sweden and its link with the large scale atmospheric circulation.Tellus, 53A(3):348–367.Chen, D., 2000. A monthly circulation climatology for Sweden and its application to a winter temperature case study. International Journal of Climatology, 20:1067–1076.
- ↑ Benestad, R., 2002. Empirically downscaled multi-model ensemble temperature and precipitation scenarios for Norway. Journal of Climate, 15:3008–3027.Benestad, R., 2004.Tentative probabilistic temperature scenarios for northern Europe.Tellus A: Dynamic Meteorology and Oceanography, 56:89–101.
- ↑ Chen, D. and X. Li, 2004. Scale dependent relationship between maximum ice extent in the Baltic Sea and atmospheric circulation. Global and Planetary Change, 41:275–283.Omstedt, A. and D. Chen, 2001. Influence of atmospheric circulation on the maximum ice extent in the Baltic Sea. Journal of Geophysical Research, 106(C3):4493–4500.
- ↑ Chen, D. and A. Omstedt, 2002. Using statistical downscaling to quantify the GCM-related uncertainty in regional climate change scenarios: A case study of Swedish precipitation. SWECLIM Newsletter, Swedish Meteorological and Hydrological Institute, Norrköping, Sweden.
- ↑ Blenckner,T. and D. Chen, 2003. Comparison of the impact of regional and north-Atlantic atmospheric circulation on an aquatic ecosystem. Climatic Research, 23:131–136.
- ↑ Hanssen-Bauer, I., E.J. Førland, J.E. Haugen and O.E.Tveito, 2003. Temperature and precipitation scenarios for Norway: Comparison of results from dynamical and empirical downscaling. Climate Research, 25:15–27.
- ↑ Hanssen-Bauer, I., 2002.Temperature and precipitation at Svalbard 1900–2050: measurements and scenarios. Polar Record, 38(206):225–232.
- ↑ Bjørge, D., J.E. Haugen and T.E. Nordeng, 2000. Future Climate in Norway. Dynamical downscaling experiments within the RegClim project. Research Report 103, Norwegian Meteorological Institute, Oslo.
- ↑ Hanssen-Bauer, I., E.J. Førland, J.E. Haugen and O.E.Tveito, 2003. Temperature and precipitation scenarios for Norway: Comparison of results from dynamical and empirical downscaling. Climate Research, 25:15–27.
- ↑ Benestad, R., 2002. Empirically downscaled multi-model ensemble temperature and precipitation scenarios for Norway. Journal of Climate, 15:3008–3027.-- Benestad, R., 2004.Tentative probabilistic temperature scenarios for northern Europe.Tellus A: Dynamic Meteorology and Oceanography, 56:89–101.
- ↑ Benestad, R., E.J. Forland and I. Hanssen-Bauer, 2002. Empirically downscaled temperature scenarios for Svalbard. Atmospheric Science Letters, 3(2–4):71–93.
- ↑ Hellström, C., D. Chen, C. Achberger and J. Räisänen, 2001. Comparison of climate change scenarios for Sweden based on statistical and dynamical downscaling of monthly precipitation. Climate Research, 19:45–55.
- ↑ Chen, D., C. Achberger, J. Räisänen and C. Hellström, 2001. Using statistical downscaling to quantify the GCM-related uncertainty in regional climate change scenarios: A case study of Swedish precipitation. SWECLIM Newsletter, Swedish Meteorological and Hydrological Institute, Norrköping, Sweden.
- ↑ Hanssen-Bauer, I., E.J. Førland, J.E. Haugen and O.E.Tveito, 2003. Temperature and precipitation scenarios for Norway: Comparison of results from dynamical and empirical downscaling. Climate Research, 25:15–27.
- ↑ Linderson, M-L., C. Achberger and D. Chen, 2004. Statistical downscaling and scenario construction of precipitation in Scania, southern Sweden. Nordic Hydrology, 35(3):261–278.
- ↑ Flato, G.M., G.J. Boer,W.G. Lee, N.A. McFarlane, D. Ramsden, M.C. Reader and A.J.Weaver, 2000.The Canadian Centre for Climate Modelling and Analysis global coupled model and its climate. Climate Dynamics, 16:451–467.
- ↑ Wilby, R.L., C.W. Dawson and E.M. Barrow, 2002. SDSM – a decision support tool for the assessment of regional climate change impacts. Environmental Modelling and Software, 17:145–157.